使用 Python 的鉛筆素描圖像
圖片在 Python 中表示為一組數(shù)字。所以我們可以進(jìn)行各種矩陣操作來(lái)得到令人興奮的結(jié)果。在本教程中,將向你展示如何只用幾行代碼創(chuàng)建“鉛筆”草圖圖像。
這個(gè)過(guò)程非常簡(jiǎn)單:
1. 灰度圖像
2. 反轉(zhuǎn)顏色
3. 模糊倒置圖像
4. 將減淡混合應(yīng)用于模糊和灰度圖像
我們可以為此選擇任何我們想要的圖像。將演示如何創(chuàng)建可以應(yīng)用于任何圖像、視頻或?qū)崟r(shí)流的對(duì)象。
導(dǎo)入庫(kù)
OpenCV 和 Numpy 是項(xiàng)目所需的唯一庫(kù)。我們使用以下兩行代碼導(dǎo)入它們:
import cv2
import numpy as np
讀取照片
這是使用 OpenCV 讀取存儲(chǔ)在磁盤(pán)上的圖像的命令之一:
frame = cv2.imread("porche.png")
此命令讀取位于當(dāng)前文件夾中的文件“image.png”,并作為幀存儲(chǔ)在內(nèi)存中。但正如我所提到的,這可以是幀序列或通過(guò)其他方法加載的圖像。
使用 OpenCV 顯示圖像
下一個(gè)重要步驟是在屏幕上顯示圖像:
cv2.imshow('image', frame)
cv2.waitKey(0)
cv2.destroyAllWindows()
圖像將在一個(gè)標(biāo)題為“image”的新窗口中打開(kāi):
灰度圖像
首先,我們需要對(duì)圖像進(jìn)行灰度處理(將其轉(zhuǎn)換為黑白)。我們可以使用 cv2 庫(kù)或 numpy.
numpy 沒(méi)有任何用于灰度的內(nèi)置函數(shù),但我們也可以很容易地將我們的圖像轉(zhuǎn)換為灰度,公式如下所示:
grayscale = np.a(chǎn)rray(np.dot(frame[..., :3], [0.299, 0.587, 0.114]), dtype=np.uint8)
grayscale = np.stack((grayscale,) * 3, axis=-1)
在這里,我們將 RGB 圖像通道與適當(dāng)?shù)闹迪喑瞬⑺鼈冞B接到單個(gè)通道。
因此,我們需要返回到 3 層圖像,使用 numpy stack 函數(shù)來(lái)實(shí)現(xiàn)。這是我們得到的灰度圖像:
反轉(zhuǎn)圖像
現(xiàn)在我們需要反轉(zhuǎn)圖像,白色應(yīng)該變成黑色。
它簡(jiǎn)單地從每個(gè)圖像像素中減去 255 。因?yàn),默認(rèn)情況下,圖像是 8 位的,最多有 256 個(gè)色調(diào):
inverted_img = 255 - grayscale
當(dāng)我們顯示反轉(zhuǎn)圖像或?qū)⑵浔4嬖诠獗P(pán)上時(shí),我們會(huì)得到以下圖片:
模糊圖像
現(xiàn)在我們需要模糊倒置的圖像。通過(guò)對(duì)倒置圖像應(yīng)用高斯濾波器來(lái)執(zhí)行模糊。這里最重要的是高斯函數(shù)或 sigma 的方差。隨著 sigma 的增加,圖像變得更模糊。Sigma 控制色散量,從而控制模糊程度?梢酝ㄟ^(guò)反復(fù)試驗(yàn)選擇合適的 sigma 值:
blur_img = cv2.GaussianBlur(inverted_img, ksize=(0, 0), sigmaX=5
模糊圖像的結(jié)果如下所示:
減淡和融合
顏色減淡和融合模式并通過(guò)降低對(duì)比度來(lái)加亮基色以反映混合色。
def dodge(self, front: np.ndarray, back: np.ndarray) -> np.ndarray:
"""The formula comes from https://en.wikipedia.org/wiki/Blend_modes
Args:
front: (np.ndarray) - front image to be applied to dodge algorithm
back: (np.ndarray) - back image to be applied to dodge algorithm
Returns:
image: (np.ndarray) - dodged image
"""
result = back*255.0 / (255.0-front)
result[result>255] = 255
result[back==255] = 255
return result.a(chǎn)stype('uint8')
final_img = self.dodge(blur_img, grayscale)
就是這樣!結(jié)果如下:
完整代碼:
class PencilSketch:
"""Apply pencil sketch effect to an image
"""
def __init__(
self,
blur_simga: int = 5,
ksize: typing.Tuple[int, int] = (0, 0),
sharpen_value: int = None,
kernel: np.ndarray = None,
) -> None:
"""
Args:
blur_simga: (int) - sigma ratio to apply for cv2.GaussianBlur
ksize: (float) - ratio to apply for cv2.GaussianBlur
sharpen_value: (int) - sharpen value to apply in predefined kernel array
kernel: (np.ndarray) - custom kernel to apply in sharpen function
"""
self.blur_simga = blur_simga
self.ksize = ksize
self.sharpen_value = sharpen_value
self.kernel = np.a(chǎn)rray([[0, -1, 0], [-1, sharpen_value,-1], [0, -1, 0]]) if kernel == None else kernel
def dodge(self, front: np.ndarray, back: np.ndarray) -> np.ndarray:
"""The formula comes from https://en.wikipedia.org/wiki/Blend_modes
Args:
front: (np.ndarray) - front image to be applied to dodge algorithm
back: (np.ndarray) - back image to be applied to dodge algorithm
Returns:
image: (np.ndarray) - dodged image
"""
result = back*255.0 / (255.0-front)
result[result>255] = 255
result[back==255] = 255
return result.a(chǎn)stype('uint8')
def sharpen(self, image: np.ndarray) -> np.ndarray:
"""Sharpen image by defined kernel size
Args:
image: (np.ndarray) - image to be sharpened
Returns:
image: (np.ndarray) - sharpened image
"""
if self.sharpen_value is not None and isinstance(self.sharpen_value, int):
inverted = 255 - image
return 255 - cv2.filter2D(src=inverted, ddepth=-1, kernel=self.kernel)
return image
def __call__(self, frame: np.ndarray) -> np.ndarray:
"""Main function to do pencil sketch
Args:
frame: (np.ndarray) - frame to excecute pencil sketch on
Returns:
frame: (np.ndarray) - processed frame that is pencil sketch type
"""
grayscale = np.a(chǎn)rray(np.dot(frame[..., :3], [0.299, 0.587, 0.114]), dtype=np.uint8)
grayscale = np.stack((grayscale,) * 3, axis=-1) # convert 1 channel grayscale image to 3 channels grayscale
inverted_img = 255 - grayscale
blur_img = cv2.GaussianBlur(inverted_img, ksize=self.ksize, sigmaX=self.blur_simga)
final_img = self.dodge(blur_img, grayscale)
sharpened_image = self.sharpen(final_img)
return sharpened_image
可以猜測(cè),除了模糊期間的blur_sigma參數(shù)外,我們沒(méi)有太多的空間可以使用。添加了一個(gè)額外的功能來(lái)銳化圖像以解決這個(gè)問(wèn)題。
它與模糊過(guò)程非常相似,只是現(xiàn)在,我們不是創(chuàng)建一個(gè)核來(lái)平均每個(gè)像素的強(qiáng)度,而是創(chuàng)建一個(gè)內(nèi)核,使像素強(qiáng)度更高,因此更容易被人眼看到。
下面是關(guān)于如何將 PencilSketch 對(duì)象用于我們的圖像的基本代碼:
# main.py
from pencilSketch import PencilSketch
from engine import Engine
if __name__ == '__main__':
pencilSketch = PencilSketch(blur_simga=5)
selfieSegmentation = Engine(image_path='data/porche.jpg', show=True, custom_objects=[pencilSketch])
selfieSegmentation.run()
結(jié)論:
這是一個(gè)非常不錯(cuò)的教程,不需要任何深入的 Python 知識(shí)就可以從任何圖像中實(shí)現(xiàn)這種“鉛筆”素描風(fēng)格。
原文標(biāo)題 : 使用 Python 的鉛筆素描圖像
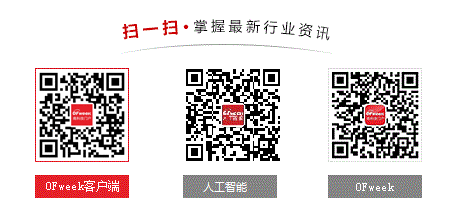
發(fā)表評(píng)論
請(qǐng)輸入評(píng)論內(nèi)容...
請(qǐng)輸入評(píng)論/評(píng)論長(zhǎng)度6~500個(gè)字
最新活動(dòng)更多
-
10月31日立即下載>> 【限時(shí)免費(fèi)下載】TE暖通空調(diào)系統(tǒng)高效可靠的組件解決方案
-
即日-11.13立即報(bào)名>>> 【在線會(huì)議】多物理場(chǎng)仿真助跑新能源汽車(chē)
-
11月28日立即報(bào)名>>> 2024工程師系列—工業(yè)電子技術(shù)在線會(huì)議
-
12月19日立即報(bào)名>> 【線下會(huì)議】OFweek 2024(第九屆)物聯(lián)網(wǎng)產(chǎn)業(yè)大會(huì)
-
即日-12.26火熱報(bào)名中>> OFweek2024中國(guó)智造CIO在線峰會(huì)
-
即日-2025.8.1立即下載>> 《2024智能制造產(chǎn)業(yè)高端化、智能化、綠色化發(fā)展藍(lán)皮書(shū)》
推薦專(zhuān)題
- 1 【一周車(chē)話】沒(méi)有方向盤(pán)和踏板的車(chē),你敢坐嗎?
- 2 特斯拉發(fā)布無(wú)人駕駛車(chē),還未迎來(lái)“Chatgpt時(shí)刻”
- 3 特斯拉股價(jià)大跌15%:Robotaxi離落地還差一個(gè)蘿卜快跑
- 4 馬斯克給的“驚喜”夠嗎?
- 5 大模型“新星”開(kāi)啟變現(xiàn)競(jìng)速
- 6 海信給AI電視打樣,12大AI智能體全面升級(jí)大屏體驗(yàn)
- 7 AI 投流卷哭創(chuàng)業(yè)者
- 8 打完“價(jià)格戰(zhàn)”,大模型還要比什么?
- 9 馬斯克致敬“國(guó)產(chǎn)蘿卜”?
- 10 神經(jīng)網(wǎng)絡(luò),誰(shuí)是盈利最強(qiáng)企業(yè)?
- 高級(jí)軟件工程師 廣東省/深圳市
- 自動(dòng)化高級(jí)工程師 廣東省/深圳市
- 光器件研發(fā)工程師 福建省/福州市
- 銷(xiāo)售總監(jiān)(光器件) 北京市/海淀區(qū)
- 激光器高級(jí)銷(xiāo)售經(jīng)理 上海市/虹口區(qū)
- 光器件物理工程師 北京市/海淀區(qū)
- 激光研發(fā)工程師 北京市/昌平區(qū)
- 技術(shù)專(zhuān)家 廣東省/江門(mén)市
- 封裝工程師 北京市/海淀區(qū)
- 結(jié)構(gòu)工程師 廣東省/深圳市