UNet分割脊柱
隨著我們每天收集更多數(shù)據(jù),人工智能(AI)將越來越多地應(yīng)用于醫(yī)療領(lǐng)域。人工智能在醫(yī)療領(lǐng)域的一個關(guān)鍵應(yīng)用是診斷。醫(yī)療診斷中的人工智能有助于決策、管理、自動化等。
脊柱是肌肉骨骼系統(tǒng)的重要組成部分,支撐著身體及其器官結(jié)構(gòu),同時在我們的活動性和負荷轉(zhuǎn)移中發(fā)揮著重要作用。它還能保護脊髓免受撞擊造成的損傷和機械沖擊。
在自動化脊柱處理管道中,脊柱標(biāo)記和分割是兩項基本任務(wù)。
可靠、準(zhǔn)確的脊柱圖像處理有望為臨床決策支持系統(tǒng)提供幫助,用于脊柱和骨骼健康的診斷、手術(shù)規(guī)劃和基于人群的分析。設(shè)計脊柱處理的自動化算法具有挑戰(zhàn)性,這主要是因為解剖學(xué)和采集協(xié)議有相當(dāng)大的差異,以及公開可用數(shù)據(jù)的嚴重短缺。
在這個博客中,我將只關(guān)注給定CT掃描數(shù)據(jù)集中脊柱的分割。標(biāo)記每一個椎骨和進一步診斷的任務(wù)沒有包含在這個博客中,可以作為這個任務(wù)的延續(xù)。
脊柱或脊柱分割是所有脊柱形態(tài)學(xué)和病理學(xué)自動量化應(yīng)用中的關(guān)鍵步驟。
隨著深度學(xué)習(xí)的到來,對于計算機斷層掃描(CT)這樣的任務(wù)來說,大而多樣的數(shù)據(jù)是一個主要的熱門資源。然而,目前還沒有一個大規(guī)模的公共數(shù)據(jù)集。
VerSe是一個大型、多探測器、多站點的CT脊柱數(shù)據(jù)集,由355名患者的374次掃描組成。2019年和2020年都有數(shù)據(jù)集。在本博客中,我將兩個數(shù)據(jù)集合并為一個數(shù)據(jù)集,以從更多數(shù)據(jù)中獲益。
這些數(shù)據(jù)是根據(jù)CC BY-SA 4.0許可證提供的,因此完全是開源的。
NIfTI(神經(jīng)成像信息技術(shù)倡議)是神經(jīng)成像的一種文件格式。NIfTI文件在神經(jīng)科學(xué)甚至神經(jīng)放射學(xué)研究的成像信息學(xué)中非常常用。每個NIfTI文件包含多達7維的元數(shù)據(jù),并支持多種數(shù)據(jù)類型。
前三個維度用于定義三個空間維度x、y和z,而第四個維度用于定義時間點t。其余維度(從第五個維度到第七個維度)用于其他用途。然而,第五維仍然可以有一些預(yù)定義的用途,例如存儲特定于體素的分布參數(shù)或保存基于向量的數(shù)據(jù)。
ITK-SNAP是一個用于在3D醫(yī)學(xué)圖像中分割結(jié)構(gòu)的軟件應(yīng)用程序。它是可以安裝在不同平臺上的開源軟件。我用它可以在3D視圖中可視化NifTi文件,以及在原始圖像上加載和覆蓋3D遮罩。我強烈建議將其用于此任務(wù)。
計算機斷層掃描(CT)是一種x射線成像程序,在該程序中,x射線以快速旋轉(zhuǎn)的速度對準(zhǔn)患者。機器收集的信號將存儲在計算機中,以生成身體的橫截面圖像,也稱為“切片”。
這些切片被稱為斷層圖像,包含比常規(guī)x射線更詳細的信息。一系列切片可以數(shù)字“疊加”在一起,形成患者的3D圖像,從而更容易識別和定位基本結(jié)構(gòu)以及可能的腫瘤或異常。
步驟如下,首先開始下載2019年和2020年的數(shù)據(jù)集。
然后,將這兩個數(shù)據(jù)集合并到它們的訓(xùn)練、驗證和測試文件夾中。下一步是讀取CT掃描圖像,并將CT掃描圖像的每個切片轉(zhuǎn)換為一系列PNG原始圖像和遮罩。后來,使用這個Github倉庫中的UNet模型,并訓(xùn)練了一個UNet模型。
數(shù)據(jù)理解:在開始數(shù)據(jù)處理和訓(xùn)練之前,我想加載幾個NIfTI文件,以便更熟悉它們的3D數(shù)據(jù)結(jié)構(gòu),能夠可視化它們并從圖像中提取元數(shù)據(jù)。
下載完VerSe數(shù)據(jù)集后,我打開了一個*.nii.gz*文件。通過讀取一個文件并查看CT掃描圖像的一個特定切片,我能夠運行Numpy transpose功能,以軸向、矢狀和冠狀三種不同視圖查看一個切片。
在對原始圖像更加熟悉,能夠從原始3D圖像中提取一個切片后,現(xiàn)在是時候查看同一切片的遮罩文件了。
正如你在下面的圖片中所看到的,能夠?qū)⒄谡智衅采w在原始圖像切片上。我們在這里看到漸變色的原因是,遮罩文件不僅存在每個脊柱的定義區(qū)域,而且它們還有不同的標(biāo)簽(用不同的顏色顯示),以及每個脊柱的編號或標(biāo)簽。為了更好地理解脊柱標(biāo)記,你可以參考本頁。
數(shù)據(jù)準(zhǔn)備:
數(shù)據(jù)準(zhǔn)備的任務(wù)是從原始圖像和遮罩文件中的每個3D CT掃描文件生成圖像切片。
它首先使用NiBabel庫讀取“.zip”格式的原始圖像和遮罩圖像,并將其轉(zhuǎn)換為Numpy數(shù)組。然后檢查每個3D圖像,檢查每個圖像的視角,并嘗試將大部分圖像轉(zhuǎn)換為矢狀視圖。
接下來,我從每個切片生成PNG文件,并將其存儲為“L”格式,即灰度值。在這種情況下,我們不需要生成RGB圖像。
在這個任務(wù)中,使用了UNet體系結(jié)構(gòu),以便能夠在數(shù)據(jù)集上應(yīng)用語義分割。為了更好地了解UNet和語義切分,建議查看這個博客。
使用了Pytorch和Pytorchvision來完成這項任務(wù)。正如提到的,這個倉庫使用PyTorch很好地實現(xiàn)了UNet,一直在使用它的一些代碼。
由于正在使用NIfTI文件,并且為了能夠在python中讀取這些文件,將使用NiBabel庫。NiBabel是一個python庫,用于讀取和寫入一些常見的醫(yī)學(xué)和神經(jīng)成像文件格式,如NIfTI文件。
Dice分數(shù):為了評估我們的模型在語義分割任務(wù)中的表現(xiàn),我們可以使用Dice分數(shù)。Dice系數(shù)是2*重疊區(qū)域(預(yù)測遮罩區(qū)域和真實遮罩區(qū)域之間)除以兩幅圖像中的像素總數(shù)。
訓(xùn)練:首先我定義了UNet類,然后定義了PyTorch數(shù)據(jù)集類,其中包括讀取和預(yù)處理圖像。預(yù)處理任務(wù)包括加載PNG文件,將它們?nèi)空{(diào)整為一個大。ㄔ诒纠袨250x250),并將它們?nèi)哭D(zhuǎn)換為NumPy數(shù)組,然后再轉(zhuǎn)換為PyTorch張量。
通過調(diào)用dataset類(VerSeDataset),我們可以在我定義的批內(nèi)準(zhǔn)備數(shù)據(jù)。為了確保原始圖像和遮罩圖像之間的映射是正確的,我調(diào)用next(iter(valid_dataloader))來獲取批次中的下一個項目并將其可視化。
后來將模型定義為model=UNet(n_channels=1,n_classes=1)。通道數(shù)是1,因為有一個灰度圖像而不是RGB,如果你的圖像是RGB圖像,你可以將n_channels改為3。類的數(shù)量是1,因為只有一個類來判斷一個像素是否是脊柱的一部分。如果你的問題是多類分割,你可以將類的數(shù)量設(shè)置為你有多少個類。
后來,訓(xùn)練了模型。對于每個批次,首先計算損失值,通過反向傳播更新參數(shù)。后來再次檢查了所有批次,只計算了驗證數(shù)據(jù)集的損失,并存儲了損失值。接下來,對train和validation的損失值進行了可視化觀察,并跟蹤了模型的性能。
保存模型后,能夠抓取其中一張圖像并將其傳遞給經(jīng)過訓(xùn)練的模型,并收到一張預(yù)測的遮罩圖像。通過將原始、真實蒙版和預(yù)測蒙版的三幅圖像并排繪制,能夠直觀地評估結(jié)果。
從上圖可以看出,模型在矢狀面和軸向視圖上都表現(xiàn)得非常好,因為預(yù)測的遮罩與真實的遮罩區(qū)域非常相似。
完整的代碼:
作者:Mazi Boustani
日期:2021年12月24日
目的:使用PyTorch訓(xùn)練UNet模型,使其能夠使用VerSe數(shù)據(jù)集分割脊柱
import numpy as np
import pandas as pd
import os
from os import listdir
from os.path import splitext
import glob
import shutil
import random
from pathlib import Path
from PIL import Image
from tqdm import tqdm
import matplotlib.pyplot as plt
%matplotlib inline
try:
import nibabel as nib
except:
raise ImportError('Install NIBABEL')
import torch
import torch.nn as nn
from torch import Tensor
import torch.nn.functional as F
from torch import optim
import torchvision.transforms as T
from torch.utils.data import DataLoader, random_split
from torch.utils.data import Dataset
# set folder paths for train and validation data
data_folder_path = "/Users/mazi/Projects/other/CT/data"
train_data = data_folder_path + "/verse_19_20_training/"
validation_data = data_folder_path + "/verse_19_20_validation/"
數(shù)據(jù)理解
# get one image to load
train_data_raw_image = train_data + "/rawdata/sub-verse521/sub-verse521_dir-ax_ct.nii.gz"
one_image = nib.load(train_data_raw_image)
# look at image shape
print(one_image.shape)
# look at image header. To understand header please refer to: https://brainder.org/2012/09/23/the-nifti-file-format/
print(one_image.header)
# look at the raw data
one_image_data = one_image.get_fdata()
print(one_image_data)
# Visualize one image in three different angles
one_image_data_axial = one_image_data
# change the view
one_image_data_sagittal = np.transpose(one_image_data, [2,1,0])
one_image_data_sagittal = np.flip(one_image_data_sagittal, axis=0)
# change the view
one_image_data_coronal = np.transpose(one_image_data, [2,0,1])
one_image_data_coronal = np.flip(one_image_data_coronal, axis=0)
fig, ax = plt.subplots(1, 3, figsize = (60, 60))
ax[0].imshow(one_image_data_axial[:,:,10], cmap ='bone')
ax[0].set_title("Axial view", fontsize=60)
ax[1].imshow(one_image_data_sagittal[:,:,260], cmap ='bone')
ax[1].set_title("Sagittal view", fontsize=60)
ax[2].imshow(one_image_data_coronal[:,:,200], cmap ='bone')
ax[2].set_title("Coronal view", fontsize=60)
plt.show()
# Overlay a mask on top of raw image (one slice of CT-scan)
train_data_mask_image = train_data + "derivatives/sub-verse521/sub-verse521_dir-ax_seg-vert_msk.nii.gz"
train_data_mask_image = nib.load(train_data_mask_image).get_fdata()
plt.figure(figsize=(10,10))
rotated_raw = np.transpose(one_image_data, [2,1,0])
rotated_raw = np.flip(rotated_raw, axis=0)
plt.imshow(rotated_raw[:,:,260], cmap ='bone', interpolation='none')
train_data_mask_image[train_data_mask_image == 0 ] = np.nan
rotated_mask = np.transpose(train_data_mask_image, [2,1,0])
rotated_mask = np.flip(rotated_mask, axis=0)
plt.imshow(rotated_mask[:,:,260], cmap ='cool')
預(yù)處理數(shù)據(jù)
# Set paths to store processed train and validation raw images and masks
processed_train = "./processed_train/"
processed_validation = "./processed_validation/"
processed_train_raw_images = processed_train + "raw_images/"
processed_train_masks = processed_train + "masks/"
processed_validation_raw_images = processed_validation + "raw_images/"
processed_validation_masks = processed_validation + "masks/"
# Read all 2019 and 2020 raw files, both train and validation
raw_train_files = glob.glob(os.path.join(train_data, 'rawdatanii.gz'))
raw_validation_files = glob.glob(os.path.join(validation_data, 'rawdatanii.gz'))
print("Raw images count train: {0}, validation: {1}".format(len(raw_train_files), len(raw_validation_
# Read all 2019 and 2020 raw files, both train and validation
raw_train_files = glob.glob(os.path.join(train_data, 'rawdatanii.gz'))
raw_validation_files = glob.glob(os.path.join(validation_data, 'rawdatanii.gz'))
print("Raw images count train: {0}, validation: {1}".format(len(raw_train_files), len(raw_validation_files)))
# Read all 2019 and 2020 derivatives files, both train and validation
masks_train_files = glob.glob(os.path.join(train_data, 'derivativesnii.gz'))
masks_validation_files = glob.glob(os.path.join(validation_data, 'derivativesnii.gz'))
print("Masks images count train: {0}, validation: {1}".format(len(masks_train_files), len(masks_validation_files)))
def read_file(nii_file):
'''
Read .nii.gz file.
Args:
nii_file (str): a file path.
Return:
3D numpy array of CT image data.
'''
return np.a(chǎn)sanyarray(nib.load(nii_file).dataobj)
def save_file(raw_data, label_data, file_name, index, output_raw_file_path, output_label_file_path):
'''
Save file into npz format.
Args:
raw_data (array): 2D numpy array of raw image data.
label_data (array): 2D numpy array of label image data.
file_name (str): file name.
index (int): slice of CT image.
output_raw_file_path (str): Path to all raw files.
output_label_file_path (str): Path to all mask files.
'''
# replace all non-zero pixels to 1
label_data = np.where(label_data > 0, 1, label_data)
unique_values = np.unique(label_data)
# if data has pixel with value of 1 means it is a positive datapoint
if len(unique_values) > 1:
raw_file_name = "{0}{1}_{2}.png".format(output_raw_file_path, file_name, index)
im = Image.fromarray(raw_data)
im = im.convert("L")
im.save(raw_file_name)
label_file_name = "{0}{1}_{2}.png".format(output_label_file_path, file_name, index)
im = Image.fromarray(label_data)
im = im.convert("L")
im.save(label_file_name)
def is_diagonal(matrix):
'''
Check if givem matrix is diagonal or not.
Args:
matrix (np array): numpy array
'''
for i in range(0, 3):
for j in range(0, 3) :
if ((i 。 j) and (matrix[i][j] != 0)):
return False
return True
def generate_data(raw_file, label_file, file_name, output_raw_file_path, output_label_file_path):
'''
Main function to read each raw and label file and generate series of images
per each slice.
Args:
raw_file (str): path to raw file.
label_file (str): path to label file.
file_name (str): file name.
output_raw_file_path (str): Path to all raw files.
output_label_file_path (str): Path to all mask files.
'''
# If skip every 2 slice. Adjacent slices can be very similar to each other and
# will generate redundant data
skip_slice = 3
continue_it = True
raw_data = read_file(raw_file)
label_data = read_file(label_file)
if "split" in raw_file:
continue_it = False
affine = nib.load(raw_file).a(chǎn)ffine
if is_diagonal(affine[:3, :3]):
transposed_raw_data = np.transpose(raw_data, [2,1,0])
transposed_raw_data = np.flip(transposed_raw_data)
transposed_label_data = np.transpose(label_data, [2,1,0])
transposed_label_data = np.flip(transposed_label_data)
else:
transposed_raw_data = np.rot90(raw_data)
transposed_raw_data = np.flip(transposed_raw_data)
transposed_label_data = np.rot90(label_data)
transposed_label_data = np.flip(transposed_label_data)
if continue_it:
if transposed_raw_data.shape:
slice_count = transposed_raw_data.shape[-1]
print("File name: ", file_name, " - Slice count: ", slice_count)
# skip some slices
for each_slice in range(1, slice_count, skip_slice):
save_file(transposed_raw_data[:,:,each_slice],
transposed_label_data[:,:,each_slice],
file_name,
each_slice,
output_raw_file_path,
output_label_file_path)
# Loop over raw images and masks and generate 'PNG' images.
print("Processing started.")
for each_raw_file in raw_train_files:
raw_file_name = each_raw_file.split("/")[-1].split("_ct.nii.gz")[0]
for each_mask_file in masks_train_files:
if raw_file_name in each_mask_file.split("/")[-1]:
generate_data(each_raw_file,
each_mask_file,
raw_file_name,
processed_train_raw_images,
processed_train_masks)
print("Processing train data done.")
# Loop over raw images and masks and generate 'PNG' images.
for each_raw_file in raw_validation_files:
raw_file_name = each_raw_file.split("/")[-1].split("_ct.nii.gz")[0]
for each_mask_file in masks_validation_files:
if raw_file_name in each_mask_file.split("/")[-1]:
generate_data(each_raw_file,
each_mask_file,
raw_file_name,
processed_validation_raw_images,
processed_validation_masks)
print("Processing validation data done.")
訓(xùn)練
# Define model parameters
DEVICE = "cuda" if torch.cuda.is_available() else "cpu"
# image size to convert to
IMAGE_HEIGHT = 250
IMAGE_WIDTH = 250
LEARNING_RATE = 1e-4
BATCH_SIZE = 10
EPOCHS = 10
NUM_WORKERS = 8
# Set the device
device = torch.device('cuda' if torch.cuda.is_available() else 'cpu')
# UNet model parts
# Source code: https://github.com/milesial/Pytorch-UNet/blob/master/unet/unet_parts.py
class DoubleConv(nn.Module):
"""(convolution => [BN] => ReLU) * 2"""
def __init__(self, in_channels, out_channels, mid_channels=None):
super().__init__()
if not mid_channels:
mid_channels = out_channels
self.double_conv = nn.Sequential(
nn.Conv2d(in_channels, mid_channels, kernel_size=3, padding=1),
nn.BatchNorm2d(mid_channels),
nn.ReLU(inplace=True),
nn.Conv2d(mid_channels, out_channels, kernel_size=3, padding=1),
nn.BatchNorm2d(out_channels),
nn.ReLU(inplace=True)
)
def forward(self, x):
return self.double_conv(x)
class Down(nn.Module):
"""Downscaling with maxpool then double conv"""
def __init__(self, in_channels, out_channels):
super().__init__()
self.maxpool_conv = nn.Sequential(
nn.MaxPool2d(2),
DoubleConv(in_channels, out_channels)
)
def forward(self, x):
return self.maxpool_conv(x)
class Up(nn.Module):
"""Upscaling then double conv"""
def __init__(self, in_channels, out_channels, bilinear=True):
super().__init__()
# if bilinear, use the normal convolutions to reduce the number of channels
if bilinear:
self.up = nn.Upsample(scale_factor=2, mode='bilinear', align_corners=True)
self.conv = DoubleConv(in_channels, out_channels, in_channels // 2)
else:
self.up = nn.ConvTranspose2d(in_channels, in_channels // 2, kernel_size=2, stride=2)
self.conv = DoubleConv(in_channels, out_channels)
def forward(self, x1, x2):
x1 = self.up(x1)
# input is CHW
diffY = x2.size()[2] - x1.size()[2]
diffX = x2.size()[3] - x1.size()[3]
x1 = F.pad(x1, [diffX // 2, diffX - diffX // 2,
diffY // 2, diffY - diffY // 2])
# if you have padding issues, see
# https://github.com/HaiyongJiang/U-Net-Pytorch-Unstructured-Buggy/commit/0e854509c2cea854e247a9c615f175f76fbb2e3a
# https://github.com/xiaopeng-liao/Pytorch-UNet/commit/8ebac70e633bac59fc22bb5195e513d5832fb3bd
x = torch.cat([x2, x1], dim=1)
return self.conv(x)
class OutConv(nn.Module):
def __init__(self, in_channels, out_channels):
super(OutConv, self).__init__()
self.conv = nn.Conv2d(in_channels, out_channels, kernel_size=1)
def forward(self, x):
return self.conv(x)
# Defining UNet architecture
# Source code: https://github.com/milesial/Pytorch-UNet/blob/master/unet/unet_model.py
class UNet(nn.Module):
def __init__(self, n_channels, n_classes, bilinear=True):
super(UNet, self).__init__(
self.n_channels = n_channels
self.n_classes = n_classes
self.bilinear = bilinear
self.inc = DoubleConv(n_channels, 64)
self.down1 = Down(64, 128)
self.down2 = Down(128, 256)
self.down3 = Down(256, 512)
factor = 2 if bilinear else 1
self.down4 = Down(512, 1024 // factor)
self.up1 = Up(1024, 512 // factor, bilinear)
self.up2 = Up(512, 256 // factor, bilinear)
self.up3 = Up(256, 128 // factor, bilinear)
self.up4 = Up(128, 64, bilinear)
self.outc = OutConv(64, n_classes)
def forward(self, x):
x1 = self.inc(x)
x2 = self.down1(x1)
x3 = self.down2(x2)
x4 = self.down3(x3)
x5 = self.down4(x4)
x = self.up1(x5, x4)
x = self.up2(x, x3)
x = self.up3(x, x2)
x = self.up4(x, x1)
logits = self.outc(x)
return logits
# Define PyTorch dataset class
# This class will access the images and masks, preprocess them for training and validation
class VerSeDataset(Dataset):
def __init__(self, raw_images_path, masks_path, images_name):
self.raw_images_path = raw_images_path
self.masks_path = masks_path
self.images_name = images_name
def __len__(self):
return len(self.images_name)
def __getitem__(self, index):
# get image and mask for a given index
img_path = os.path.join(self.raw_images_path, self.images_name[index])
mask_path = os.path.join(self.masks_path, self.images_name[index])
# read the image and mask
image = Image.open(img_path)
mask = Image.open(mask_path)
# resize image and change the shape to (1, image_width, image_height)
w, h = image.size
image = image.resize((w, h), resample=Image.BICUBIC)
image = T.Resize(size=(IMAGE_WIDTH, IMAGE_HEIGHT))(image)
image_ndarray = np.a(chǎn)sarray(image)
image_ndarray = image_ndarray.reshape(1, image_ndarray.shape[0], image_ndarray.shape[1])
# resize the mask. Mask shape is (image_width, image_height)
mask = mask.resize((w, h), resample=Image.NEAREST)
mask = T.Resize(size=(IMAGE_WIDTH, IMAGE_HEIGHT))(mask)
mask_ndarray = np.a(chǎn)sarray(mask)
return {
'image': torch.a(chǎn)s_tensor(image_ndarray.copy()).float().contiguous(),
'mask': torch.a(chǎn)s_tensor(mask_ndarray.copy()).float().contiguous(
}
# Get path for all images and masks
train_images_paths = os.listdir(processed_train_raw_images)
train_masks_paths = os.listdir(processed_train_masks)
validation_images_paths = os.listdir(processed_validation_raw_images)
validation_masks_paths = os.listdir(processed_validation_masks)
# Load both images and masks data
train_data = VerSeDataset(processed_train_raw_images, processed_train_masks, train_images_paths)
valid_data = VerSeDataset(processed_validation_raw_images, processed_validation_masks, validation_images_paths)
# Create PyTorch DataLoader
train_dataloader = DataLoader(train_data, batch_size=BATCH_SIZE, shuffle=True)
valid_dataloader = DataLoader(valid_data, batch_size=BATCH_SIZE, shuffle=False)
# Looking at one image and mask from one batch just to check them visually
next_image = next(iter(valid_dataloader))
fig, ax = plt.subplots(1, 2, figsize = (60, 60))
ax[0].imshow(next_image['image'][0][0,:,:], cmap ='bone')
ax[0].set_title("Raw image", fontsize=60)
ax[1].imshow(next_image['mask'][0][:,:], cmap ='bone')
ax[1].set_title("Mask image", fontsize=60)
plt.show()
# Defining Dice loss class
# Source code: https://www.kaggle.com/bigironsphere/loss-function-library-keras-pytorch
class DiceLoss(nn.Module):
def __init__(self, weight=None, size_average=True):
super(DiceLoss, self).__init__()
def forward(self, inputs, targets, smooth=1):
inputs = torch.sigmoid(inputs)
# flatten label and prediction tensors
inputs = inputs.view(-1)
targets = targets.view(-1)
intersection = (inputs * targets).sum()
dice = (2.*intersection + smooth)/(inputs.sum() + targets.sum() + smooth)
bce = F.binary_cross_entropy_with_logits(inputs, targets)
pred = torch.sigmoid(inputs)
loss = bce * 0.5 + dice * (1 - 0.5)
# subtract 1 to calculate loss from dice value
return 1 - dice
# Define model as UNet
model = UNet(n_channels=1, n_classes=1)
model.to(device=device)
optimizer = optim.Adam(model.parameters(), lr=LEARNING_RATE)
# Train and validate
train_loss = []
val_loss = []
for epoch in range(EPOCHS):
model.train()
train_running_loss = 0.0
counter = 0
with tqdm(total=len(train_data), desc=f'Epoch {epoch + 1}/{EPOCHS}', unit='img') as pbar:
for batch in train_dataloader:
counter+=1
image = batch['image'].to(DEVICE)
mask = batch['mask'].to(DEVICE)
optimizer.zero_grad()
outputs = model(image)
outputs = outputs.squeeze(1)
loss = DiceLoss()(outputs, mask)
train_running_loss += loss.item()
loss.backward()
optimizer.step()
pbar.update(image.shape[0])
pbar.set_postfix(**{'loss (batch)': loss.item()})
train_loss.a(chǎn)ppend(train_running_loss/counter)
model.eval()
valid_running_loss = 0.0
counter = 0
with torch.no_grad():
for i, data in enumerate(valid_dataloader):
counter += 1
image = data['image'].to(DEVICE)
mask = data['mask'].to(DEVICE)
outputs = model(image)
outputs = outputs.squeeze(1)
loss = DiceLoss()(outputs, mask)
valid_running_loss += loss.item()
val_loss.a(chǎn)ppend(valid_running_loss)
Epoch 1/10: 100%|██████████| 4790/4790 [4:00:34<00:00, 3.01s/img, loss (batch)=0.385]
Epoch 2/10: 100%|██████████| 4790/4790 [4:00:02<00:00, 3.01s/img, loss (batch)=0.268]
Epoch 3/10: 100%|██████████| 4790/4790 [3:57:30<00:00, 2.98s/img, loss (batch)=0.152]
Epoch 4/10: 100%|██████████| 4790/4790 [3:57:05<00:00, 2.97s/img, loss (batch)=0.105]
Epoch 5/10: 100%|██████████| 4790/4790 [4:08:29<00:00, 3.11s/img, loss (batch)=0.103]
Epoch 6/10: 100%|██████████| 4790/4790 [4:04:12<00:00, 3.06s/img, loss (batch)=0.0874]
Epoch 7/10: 100%|██████████| 4790/4790 [4:02:00<00:00, 3.03s/img, loss (batch)=0.0759]
Epoch 8/10: 100%|██████████| 4790/4790 [3:58:32<00:00, 2.99s/img, loss (batch)=0.0655]
Epoch 9/10: 100%|██████████| 4790/4790 [4:00:47<00:00, 3.02s/img, loss (batch)=0.0644]
Epoch 10/10: 100%|██████████| 4790/4790 [4:08:54<00:00, 3.12s/img, loss (batch)=0.0604]
# Plot train vs validation loss
plt.figure(figsize=(10, 7))
plt.plot(train_loss, color="orange", label='train loss')
plt.plot(val_loss, color="red", label='validation loss')
plt.xlabel("Epochs")
plt.ylabel("Loss")
plt.legend()
plt.show()
# Save the trained model
torch.save({
'epoch': EPOCHS,
'model_state_dict': model.state_dict(),
'optimizer_state_dict': optimizer.state_dict(),
}, "./unet_model.pth")
# Visually look at one prediction
next_image = next(iter(valid_dataloader))
# do predict
outputs = model(next_image['image'].float())
outputs = outputs.detach().cpu()
loss = DiceLoss()(outputs, next_image['mask'])
print("Dice Score: ", 1- loss.item())
outputs[outputs<=0.0] = 0
outputs[outputs>0.0] = 1.0
# plot all three images
fig, ax = plt.subplots(1, 3, figsize = (60, 60))
ax[0].imshow(next_image['image'][0][0,:,:], cmap ='bone')
ax[0].set_title("Raw Image", fontsize=60)
ax[1].imshow(next_image['mask'][0][:,:], cmap ='bone')
ax[1].set_title("True Mask", fontsize=60)
ax[2].imshow(outputs[0,0,:,:], cmap ='bone')
ax[2].set_title("Predicted Mask", fontsize=60)
plt.show()
未來的工作:這個任務(wù)也可以用3D UNet完成,這可能是學(xué)習(xí)脊柱結(jié)構(gòu)的更好方法。
因為我們對每個椎骨的每個遮罩區(qū)域都有標(biāo)簽,所以我們可以進一步進行多類遮罩分割。此外,當(dāng)圖像視圖為矢狀視圖時,模型性能最好,因此,將所有切片轉(zhuǎn)換為矢狀視圖可能會得到更好的結(jié)果。
感謝閱讀!
原文標(biāo)題 : UNet分割脊柱
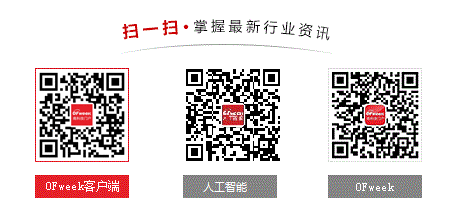
請輸入評論內(nèi)容...
請輸入評論/評論長度6~500個字
最新活動更多
-
即日-11.13立即報名>>> 【在線會議】多物理場仿真助跑新能源汽車
-
11月28日立即報名>>> 2024工程師系列—工業(yè)電子技術(shù)在線會議
-
12月19日立即報名>> 【線下會議】OFweek 2024(第九屆)物聯(lián)網(wǎng)產(chǎn)業(yè)大會
-
即日-12.26火熱報名中>> OFweek2024中國智造CIO在線峰會
-
即日-2025.8.1立即下載>> 《2024智能制造產(chǎn)業(yè)高端化、智能化、綠色化發(fā)展藍皮書》
-
精彩回顧立即查看>> 【限時免費下載】TE暖通空調(diào)系統(tǒng)高效可靠的組件解決方案
推薦專題
-
5 夾縫中的文遠知行
- 高級軟件工程師 廣東省/深圳市
- 自動化高級工程師 廣東省/深圳市
- 光器件研發(fā)工程師 福建省/福州市
- 銷售總監(jiān)(光器件) 北京市/海淀區(qū)
- 激光器高級銷售經(jīng)理 上海市/虹口區(qū)
- 光器件物理工程師 北京市/海淀區(qū)
- 激光研發(fā)工程師 北京市/昌平區(qū)
- 技術(shù)專家 廣東省/江門市
- 封裝工程師 北京市/海淀區(qū)
- 結(jié)構(gòu)工程師 廣東省/深圳市