使用U-Net方法對航空圖像進行語義分割
在機器學(xué)習(xí)中,模型是在各種應(yīng)用中訓(xùn)練的,特別是在深度學(xué)習(xí)和圖像數(shù)據(jù)集上;诰矸e運算的方法在許多領(lǐng)域都進行了研究,尤其是手臂檢測、自動駕駛汽車、無人機航拍圖像、戰(zhàn)爭技術(shù)。人眼能夠很容易地對所看到的進行分類和區(qū)分。然而,在人工智能技術(shù)中,這種能力的等價物,即理解圖像的問題,屬于計算機視覺領(lǐng)域。顧名思義,計算機視覺是以計算機可以理解的方式引入(分類)圖像,下一步是使用不同的方法對這些圖像進行操作。本文介紹了一種分割方法,即U-Net體系結(jié)構(gòu),該體系結(jié)構(gòu)是為生物醫(yī)學(xué)圖像分割而開發(fā)的,并包括一個實際項目,該項目使用U-Net對無人機捕獲的航空圖像進行分割。
目錄
1.語義分割
2.U-Net架構(gòu)
3.教程
3.1. 數(shù)據(jù)預(yù)處理
3.2. 基于U-Net的語義分割
3.3. 基于遷移學(xué)習(xí)的U-Net語義分割
4.結(jié)論
5.參考文獻
語義分割
圖像是由數(shù)字組成的像素矩陣。在圖像處理技術(shù)中,對這些數(shù)字進行一些調(diào)整,然后以不同的方式表示圖像,并使其適合相關(guān)研究或解釋。
卷積過程是一種基本的數(shù)學(xué)像素運算,它提供了從不同角度評估圖像的機會。
例如,可以使用濾波器進行圖像的邊緣檢測,也可以通過將圖像從RGB格式轉(zhuǎn)換為灰度,從不同角度解釋和使用圖像。
基于深度學(xué)習(xí)模型和卷積層,人們對圖像內(nèi)容進行了更全面的研究,如特征提取和分類。
如上圖所示,使用邊界框檢測圖像內(nèi)容中的對象稱為對象檢測。
語義分割是一種逐像素的標(biāo)記操作,它用一個標(biāo)簽來顯示圖片中相同類型的對象(天空、貓、狗、人、路、車、山、海等),即顏色。
即時分割是指每個實例都被單獨標(biāo)記,通過以不同的顏色顯示來分隔每個對象。
如上所述,在這些操作的背景下,針對不同的用途開發(fā)了各種彼此不同的CNN模型和復(fù)雜模型。PSPNet、DeepLab、LinkNet、U-Net、Mask R-CNN就是其中的一些模型。我們可以說,分割過程是基于機器學(xué)習(xí)的應(yīng)用(如自動駕駛汽車)項目的核心。
總之,計算機視覺中的語義分割是一種基于像素的標(biāo)記方法。如果相同類型的對象用單一顏色表示,則稱為語義分割;如果每個對象用唯一的顏色(標(biāo)簽)表示,則稱為實例分割。
U-Net體系結(jié)構(gòu)
U-Net是一種特定類型的卷積神經(jīng)網(wǎng)絡(luò)架構(gòu),2015年在德國弗萊堡大學(xué)計算機科學(xué)系和生物信號研究中心為生物醫(yī)學(xué)圖像(計算機斷層掃描、顯微圖像、MRI掃描等)開發(fā)。
當(dāng)我們考慮技術(shù)思想時,該模型由編碼器和解碼器組成,編碼器(收縮)是下采樣(主要是遷移學(xué)習(xí)中的預(yù)訓(xùn)練權(quán)重),解碼器(提。┦巧喜蓸硬糠郑幻麨閁-Net,因為它的方案是U形的,如下圖所示。該模型可根據(jù)不同的研究進行配置。
在以下教程中,為航空圖像的語義分割配置了U-Net模型,如下所示:
# -*- coding: utf-8 -*-
"""
@author: Ibrahim Kovan
https://ibrahimkovan.medium.com/
"""
from tensorflow.keras.models import Model
from tensorflow.keras.layers import Input, Conv2D, MaxPooling2D, UpSampling2D, concatenate, Conv2DTranspose, BatchNormalization, Dropout, Lambda
from tensorflow.keras import backend as K
def multiclass_unet_architecture(n_classes=2, height=256, width=256, channels=3):
inputs = Input((height, width, channels))
#Contraction path
conv_1 = Conv2D(16, (3, 3), activation='relu', kernel_initializer='he_normal', padding='same')(inputs)
conv_1 = Dropout(0.1)(conv_1)
conv_1 = Conv2D(16, (3, 3), activation='relu', kernel_initializer='he_normal', padding='same')(conv_1)
pool_1 = MaxPooling2D((2, 2))(conv_1)
conv_2 = Conv2D(32, (3, 3), activation='relu', kernel_initializer='he_normal', padding='same')(pool_1)
conv_2 = Dropout(0.1)(conv_2)
conv_2 = Conv2D(32, (3, 3), activation='relu', kernel_initializer='he_normal', padding='same')(conv_2)
pool_2 = MaxPooling2D((2, 2))(conv_2)
conv_3 = Conv2D(64, (3, 3), activation='relu', kernel_initializer='he_normal', padding='same')(pool_2)
conv_3 = Dropout(0.1)(conv_3)
conv_3 = Conv2D(64, (3, 3), activation='relu', kernel_initializer='he_normal', padding='same')(conv_3)
pool_3 = MaxPooling2D((2, 2))(conv_3)
conv_4 = Conv2D(128, (3, 3), activation='relu', kernel_initializer='he_normal', padding='same')(pool_3)
conv_4 = Dropout(0.1)(conv_4)
conv_4 = Conv2D(128, (3, 3), activation='relu', kernel_initializer='he_normal', padding='same')(conv_4)
pool_4 = MaxPooling2D(pool_size=(2, 2))(conv_4)
conv_5 = Conv2D(256, (3, 3), activation='relu', kernel_initializer='he_normal', padding='same')(pool_4)
conv_5 = Dropout(0.2)(conv_5)
conv_5 = Conv2D(256, (3, 3), activation='relu', kernel_initializer='he_normal', padding='same')(conv_5)
#Expansive path
u6 = Conv2DTranspose(128, (2, 2), strides=(2, 2), padding='same')(conv_5)
u6 = concatenate([u6, conv_4])
conv_6 = Conv2D(128, (3, 3), activation='relu', kernel_initializer='he_normal', padding='same')(u6)
conv_6 = Dropout(0.2)(conv_6)
conv_6 = Conv2D(128, (3, 3), activation='relu', kernel_initializer='he_normal', padding='same')(conv_6)
u7 = Conv2DTranspose(64, (2, 2), strides=(2, 2), padding='same')(conv_6)
u7 = concatenate([u7, conv_3])
conv_7 = Conv2D(64, (3, 3), activation='relu', kernel_initializer='he_normal', padding='same')(u7)
conv_7 = Dropout(0.1)(conv_7)
conv_7 = Conv2D(64, (3, 3), activation='relu', kernel_initializer='he_normal', padding='same')(conv_7)
u8 = Conv2DTranspose(32, (2, 2), strides=(2, 2), padding='same')(conv_7)
u8 = concatenate([u8, conv_2])
conv_8 = Conv2D(32, (3, 3), activation='relu', kernel_initializer='he_normal', padding='same')(u8)
conv_8 = Dropout(0.2)(conv_8)
conv_8 = Conv2D(32, (3, 3), activation='relu', kernel_initializer='he_normal', padding='same')(conv_8)
u9 = Conv2DTranspose(16, (2, 2), strides=(2, 2), padding='same')(conv_8)
u9 = concatenate([u9, conv_1], axis=3)
conv_9 = Conv2D(16, (3, 3), activation='relu', kernel_initializer='he_normal', padding='same')(u9)
conv_9 = Dropout(0.1)(conv_9)
conv_9 = Conv2D(16, (3, 3), activation='relu', kernel_initializer='he_normal', padding='same')(conv_9)
outputs = Conv2D(n_classes, (1, 1), activation='softmax')(conv_9)
model = Model(inputs=[inputs], outputs=[outputs])
model.summary()
return model
def jacard(y_true, y_pred):
y_true_c = K.flatten(y_true)
y_pred_c = K.flatten(y_pred)
intersection = K.sum(y_true_c * y_pred_c)
return (intersection + 1.0) / (K.sum(y_true_c) + K.sum(y_pred_c) - intersection + 1.0)
def jacard_loss(y_true, y_pred):
return -jacard(y_true,y_pred)
如果我們采用上述代碼塊:
1、輸入定義為256x256x3。
2、使用16個濾波器的conv_1,可獲得256x256x16的輸出。使用pool_1中的Maxpooling,它將減少到128x128x16。
3、使用32個濾波器的conv_2,可獲得256x256x32的輸出。使用pool_2,可獲得64x64x32的輸出。
4、使用64個濾波器的conv_3,可獲得64x64x64的輸出。使用pool_3,可獲得32x32x64的輸出。
5、使用128個濾波器的conv_4,可獲得32x32x128的輸出。使用pool_4,可獲得16x16x128的輸出。
6、使用256個濾波器的conv_5,可獲得16x16x256的輸出,并從此點開始進行上采樣。在濾波器數(shù)量為128和(2x2)的u6中,conv_5通過Conv2DTranspose和級聯(lián)得到32x32x128的輸出,級聯(lián)通過u6、conv_4執(zhí)行。因此,u6輸出為32x32x256。使用帶有128個濾波器的conv_6,它將變?yōu)?2x32x128。
7、濾波器數(shù)量為64且(2x2)的u7通過應(yīng)用于conv_6并將u7與conv_3串聯(lián),變?yōu)?4x64x64。此操作的結(jié)果是,u7被定義為64x64x128,并在conv_7中變?yōu)?4x64x64。
8、濾波器數(shù)量為32和(2x2)的u8通過應(yīng)用于conv_7并將u7與conv_2串聯(lián),變?yōu)?28x128x32。此操作的結(jié)果是,u8被定義為128x128x64,并使用conv_8變?yōu)?28x128x32。
9、通過應(yīng)用于conv_8并將u9與conv_1串聯(lián),濾波器數(shù)量為16和(2x2)的u9變?yōu)?56x256x16。此操作的結(jié)果是,u9被定義為256x256x32,并通過conv_9變?yōu)?56x256x16。
10、輸出使用softmax激活完成分類過程,最終輸出采用256x256x1的形式。
使用不同速率的dropout來防止過擬合。
教程
在編碼部分,可以使用不同的方法對數(shù)據(jù)集進行訓(xùn)練。
在本研究中,RGB(原始圖像)數(shù)據(jù)集定義為x,并且該模型是通過使用真實標(biāo)簽(分割標(biāo)記圖像)作為y來訓(xùn)練的。在未來的文章中,還將討論使用掩碼數(shù)據(jù)集的方法。
RGB圖像和標(biāo)簽如下圖所示。該研究旨在用這種方法訓(xùn)練數(shù)據(jù)集,并使外部呈現(xiàn)的圖像能夠像訓(xùn)練數(shù)據(jù)一樣進行分割。
它關(guān)注的是編碼體系結(jié)構(gòu)部分,而不是實現(xiàn)高性能。這是由于使用圖像數(shù)據(jù)集時涉及的計算復(fù)雜性。
例如,雖然原始圖像為6000x4000像素,但已將其轉(zhuǎn)換為256x256像素以避免計算復(fù)雜性。通過這些操作,目的是通過放棄準(zhǔn)確性來確保編碼體系結(jié)構(gòu)正常工作。
數(shù)據(jù)預(yù)處理
# -*- coding: utf-8 -*-
"""
@author: Ibrahim Kovan
https://ibrahimkovan.medium.com/
dataset: http://www.dronedataset.icg.tugraz.a(chǎn)t/
dataset link: https://www.kaggle.com/awsaf49/semantic-drone-dataset
License: CC0: Public Domain
"""
#%% Libraries
"""1"""
from architecture import multiclass_unet_architecture, jacard, jacard_loss
from tensorflow.keras.utils import normalize
import os
import glob
import cv2
import numpy as np
from matplotlib import pyplot as plt
import random
from skimage.io import imshow
from PIL import Image
import pandas as pd
from sklearn.preprocessing import MinMaxScaler, StandardScaler
from tensorflow.keras.utils import to_categorical
from sklearn.model_selection import train_test_split
import segmentation_models as sm
from tensorflow.keras.metrics import MeanIoU
#%% Import train and mask dataset
"""2"""
train_path = r"C:UsersibrahDesktopU-Netdataset raining_setimages.jpg"
def importing_data(path):
sample = []
for filename in glob.glob(path):
img = Image.open(filename,'r')
img = img.resize((256,256))
img = np.a(chǎn)rray(img)
sample.a(chǎn)ppend(img)
return sample
data_train = importing_data(train_path)
data_train = np.a(chǎn)sarray(data_train)
mask_path = r"C:UsersibrahDesktopU-Netdataset raining_setgtsemanticlabel_images.png"
def importing_data(path):
sample = []
for filename in glob.glob(path):
img = Image.open(filename,'r')
img = img.resize((256,256))
img = np.a(chǎn)rray(img)
sample.a(chǎn)ppend(img)
return sample
data_mask = importing_data(mask_path)
data_mask = np.a(chǎn)sarray(data_mask)
#%% Random visualization
x = random.randint(0, len(data_train))
plt.figure(figsize=(24,18))
plt.subplot(1,2,1)
imshow(data_train[x])
plt.subplot(1,2,2)
imshow(data_mask[x])
plt.show()
#%% Normalization
"""3"""
scaler = MinMaxScaler()
nsamples, nx, ny, nz = data_train.shape
d2_data_train = data_train.reshape((nsamples,nx*ny*nz))
train_images = scaler.fit_transform(d2_data_train)
train_images = train_images.reshape(400,256,256,3)
#%% Labels of the masks
"""4"""
labels = pd.read_csv(r"C:UsersibrahDesktopU-Netdataset raining_setgtsemantic/class_dict.csv")
labels = labels.drop(['name'],axis = 1)
labels = np.a(chǎn)rray(labels)
def image_labels(label):
image_labels = np.zeros(label.shape, dtype=np.uint8)
for i in range(24):
image_labels [np.a(chǎn)ll(label == labels[i,:],axis=-1)] = i
image_labels = image_labels[:,:,0]
return image_labels
label_final = []
for i in range(data_mask.shape[0]):
label = image_labels(data_mask[i])
label_final.a(chǎn)ppend(label)
label_final = np.a(chǎn)rray(label_final)
#%% train_test
"""5"""
n_classes = len(np.unique(label_final))
labels_cat = to_categorical(label_final, num_classes=n_classes)
x_train, x_test, y_train, y_test = train_test_split(train_images, labels_cat, test_size = 0.20, random_state = 42)
1-導(dǎo)入庫。從architecture import multiclass_unet_architecture中,定義了jacard,jacard_loss,并從上述部分導(dǎo)入。
2-6000x4000像素的RGB原始圖像和相應(yīng)標(biāo)簽的大小調(diào)整為256x256像素。
3-MinMaxScaler用于縮放RGB圖像。
4-導(dǎo)入真實標(biāo)簽。數(shù)據(jù)集中有23個標(biāo)簽,并根據(jù)像素值將標(biāo)簽分配給圖像。
5-標(biāo)簽數(shù)據(jù)集是用于分類的one-h(huán)ot編碼數(shù)據(jù)集,數(shù)據(jù)被分離為訓(xùn)練集和測試集。
使用U-Net的語義分割(從頭開始)
#%% U-Net
"""6"""
img_height = x_train.shape[1]
img_width = x_train.shape[2]
img_channels = x_train.shape[3]
metrics=['accuracy', jacard]
def get_model():
return multiclass_unet_architecture(n_classes=n_classes, height=img_height,
width=img_width, channels=img_channels)
model = get_model()
model.compile(optimizer='adam', loss='categorical_crossentropy', metrics=metrics)
model.summary()
history = model.fit(x_train, y_train,
batch_size = 16,
verbose=1,
epochs=100,
validation_data=(x_test, y_test),
shuffle=False)
#%%
"""7"""
loss = history.history['loss']
val_loss = history.history['val_loss']
epochs = range(1, len(loss) + 1)
plt.plot(epochs, loss, 'y', label='Training loss')
plt.plot(epochs, val_loss, 'r', label='Validation loss')
plt.title('Training and validation loss')
plt.xlabel('Epochs')
plt.ylabel('Loss')
plt.legend()
plt.show()
acc = history.history['jacard']
val_acc = history.history['val_jacard']
plt.plot(epochs, acc, 'y', label='Training Jaccard')
plt.plot(epochs, val_acc, 'r', label='Validation Jaccard')
plt.title('Training and validation Jacard')
plt.xlabel('Epochs')
plt.ylabel('Jaccard')
plt.legend()
plt.show()
#%%
"""8"""
y_pred=model.predict(x_test)
y_pred_argmax=np.a(chǎn)rgmax(y_pred, axis=3)
y_test_argmax=np.a(chǎn)rgmax(y_test, axis=3)
test_jacard = jacard(y_test,y_pred)
print(test_jacard)
#%%
"""9"""
fig, ax = plt.subplots(5, 3, figsize = (12,18))
for i in range(0,5):
test_img_number = random.randint(0, len(x_test))
test_img = x_test[test_img_number]
ground_truth=y(tǒng)_test_argmax[test_img_number]
test_img_input=np.expand_dims(test_img, 0)
prediction = (model.predict(test_img_input))
predicted_img=np.a(chǎn)rgmax(prediction, axis=3)[0,:,:]
ax[i,0].imshow(test_img)
ax[i,0].set_title("RGB Image",fontsize=16)
ax[i,1].imshow(ground_truth)
ax[i,1].set_title("Ground Truth",fontsize=16)
ax[i,2].imshow(predicted_img)
ax[i,2].set_title("Prediction",fontsize=16)
i+=i
plt.show()
6-在訓(xùn)練過程中使用準(zhǔn)確度和Jaccard指數(shù)。優(yōu)化器設(shè)置為“adam”,損失設(shè)置為“categorical_crossentropy”,因為它只是一個復(fù)雜的分類問題。該模型配備了這些設(shè)置。
7-val_jaccard和訓(xùn)練過程的損失是可視化的。下圖顯示了val_jaccard。
8-測試數(shù)據(jù)集的Jaccard系數(shù)計算為0.5532。
9-從測試數(shù)據(jù)集中選擇5幅隨機圖像,用訓(xùn)練好的算法進行預(yù)測,結(jié)果如下圖所示。
基于遷移學(xué)習(xí)的U-Net語義分割
#%% pre-trained model
"""10"""
BACKBONE = 'resnet34'
preprocess_input = sm.get_preprocessing(BACKBONE)
# preprocess input
x_train_new = preprocess_input(x_train)
x_test_new = preprocess_input(x_test)
# define model
model_resnet_backbone = sm.Unet(BACKBONE, encoder_weights='imagenet', classes=n_classes, activation='softmax')
metrics=['accuracy', jacard]
# compile keras model with defined optimozer, loss and metrics
#model_resnet_backbone.compile(optimizer='adam', loss=focal_loss, metrics=metrics)
model_resnet_backbone.compile(optimizer='adam', loss='categorical_crossentropy', metrics=metrics)
print(model_resnet_backbone.summary())
history_tf=model_resnet_backbone.fit(x_train_new,
y_train,
batch_size=16,
epochs=100,
verbose=1,
validation_data=(x_test_new, y_test))
#%%
"""11"""
history = history_tf
loss = history.history['loss']
val_loss = history.history['val_loss']
epochs = range(1, len(loss) + 1)
plt.plot(epochs, loss, 'y', label='Training loss')
plt.plot(epochs, val_loss, 'r', label='Validation loss')
plt.title('Training and validation loss')
plt.xlabel('Epochs')
plt.ylabel('Loss')
plt.legend()
plt.show()
acc = history.history['jacard']
val_acc = history.history['val_jacard']
plt.plot(epochs, acc, 'y', label='Training IoU')
plt.plot(epochs, val_acc, 'r', label='Validation IoU')
plt.title('Training and validation Jaccard')
plt.xlabel('Epochs')
plt.ylabel('Jaccard')
plt.legend()
plt.show()
#%%
"""12"""
y_pred_tf=model_resnet_backbone.predict(x_test)
y_pred_argmax_tf=np.a(chǎn)rgmax(y_pred_tf, axis=3)
y_test_argmax_tf=np.a(chǎn)rgmax(y_test, axis=3)
test_jacard = jacard(y_test,y_pred_tf)
print(test_jacard)
#%%
"""13"""
fig, ax = plt.subplots(5, 3, figsize = (12,18))
for i in range(0,5):
test_img_number = random.randint(0, len(x_test))
test_img_tf = x_test_new[test_img_number]
ground_truth_tf=y(tǒng)_test_argmax_tf[test_img_number]
test_img_input_tf=np.expand_dims(test_img_tf, 0)
prediction_tf = (model_resnet_backbone.predict(test_img_input_tf))
predicted_img_transfer_learning=np.a(chǎn)rgmax(prediction_tf, axis=3)[0,:,:]
ax[i,0].imshow(test_img_tf)
ax[i,0].set_title("RGB Image",fontsize=16)
ax[i,1].imshow(ground_truth_tf)
ax[i,1].set_title("Ground Truth",fontsize=16
ax[i,2].imshow(predicted_img_transfer_learning)
ax[i,2].set_title("Prediction(Transfer Learning)",fontsize=16)
i+=i
plt.show()
10-使用resnet34重新準(zhǔn)備數(shù)據(jù)集。將“Adam”設(shè)置為優(yōu)化器,“categorical_crossentropy”設(shè)置為損失函數(shù),并對模型進行訓(xùn)練。
11-val_jaccard和訓(xùn)練過程的丟失是可視化的。下圖展示了val_jaccard。
12-測試數(shù)據(jù)集的Jaccard索引值計算為0.6545。
13-從測試數(shù)據(jù)集中選擇5幅隨機圖像,用訓(xùn)練好的算法進行預(yù)測,結(jié)果如下圖所示。
結(jié)論
本文提出了一種基于U-Net的衛(wèi)星圖像語義分割方法,該方法是為生物醫(yī)學(xué)圖像分割而開發(fā)的。
本研究考慮了兩種主要方法。第一種方法涉及使用從頭開始的實現(xiàn)來訓(xùn)練配置的u-net模型。第二種方法涉及使用遷移學(xué)習(xí)技術(shù)訓(xùn)練模型,即預(yù)訓(xùn)練權(quán)重。
在實現(xiàn)部分,對相應(yīng)的帶有真實標(biāo)簽的圖像進行one-h(huán)ot編碼,并對模型進行分類訓(xùn)練。Jaccard系數(shù)作為度量。
"""14"""
fig, ax = plt.subplots(5, 4, figsize = (16,20))
for i in range(0,5):
test_img_number = random.randint(0, len(x_test))
test_img = x_test[test_img_number]
ground_truth=y(tǒng)_test_argmax[test_img_number]
test_img_input=np.expand_dims(test_img, 0)
prediction = (model.predict(test_img_input))
predicted_img=np.a(chǎn)rgmax(prediction, axis=3)[0,:,:]
test_img_tf = x_test_new[test_img_number]
ground_truth_tf=y(tǒng)_test_argmax_tf[test_img_number]
test_img_input_tf=np.expand_dims(test_img_tf, 0)
prediction_tf = (model_resnet_backbone.predict(test_img_input_tf))
predicted_img_transfer_learning=np.a(chǎn)rgmax(prediction_tf, axis=3)[0,:,:]
ax[i,0].imshow(test_img_tf)
ax[i,0].set_title("RGB Image",fontsize=16)
ax[i,1].imshow(ground_truth_tf)
ax[i,1].set_title("Ground Truth",fontsize=16)
ax[i,2].imshow(predicted_img)
ax[i,2].set_title("Prediction",fontsize=16)
ax[i,3].imshow(predicted_img_transfer_learning)
ax[i,3].set_title("Prediction Transfer Learning",fontsize=16)
i+=i
plt.show()
調(diào)整大小并不推薦,因為分割操作中的大小更改會出現(xiàn)不希望的偏移,但由于計算復(fù)雜性,數(shù)據(jù)集從6000x4000調(diào)整為256x256。因此,模型的成功率極低。防止這種情況的一些主要措施是使用高分辨率數(shù)據(jù)集和/或使用修補。
使用調(diào)整大小的數(shù)據(jù)集,評估了兩種不同的方法,結(jié)果如圖8所示。從Jaccard索引值來看,遷移學(xué)習(xí)方法得到的值為0.6545,而scratch構(gòu)建模型得到的值為0.5532?梢钥闯觯褂妙A(yù)訓(xùn)練模型得到的分割過程更為成功。
在以后的文章中,不同的編碼方法將涵蓋不同的方法。
參考引用
O. Ronneberger, P. Fischer, and T. Brox, “LNCS 9351 — U-Net: Convolutional Networks for Biomedical Image Segmentation,” 2015, doi: 10.1007/978–3–319–24574–4_28.
A. Arnab et al., “Conditional Random Fields Meet Deep Neural Networks for Semantic Segmentation,” IEEE Signal Process. Mag., vol. XX, 2018.
J. Y. C. Chen, G. F. Eds, and G. Goos, 2020_Book_VirtualAugmentedAndMixedReality. 2020.
J. Maurya, R. Hebbalaguppe, and P. Gupta, “Real-Time Hand Segmentation on Frugal Head-mounted Device for Gestural Interface,” Proc. — Int. Conf. Image Process. ICIP, pp. 4023–4027, 2018, doi: 10.1109/ICIP.2018.8451213.
原文標(biāo)題 : 使用U-Net方法對航空圖像進行語義分割
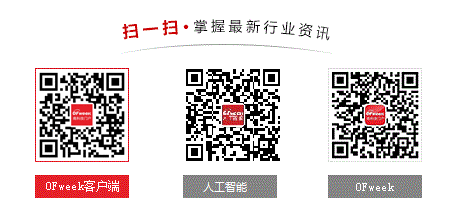
請輸入評論內(nèi)容...
請輸入評論/評論長度6~500個字
最新活動更多
-
10月31日立即下載>> 【限時免費下載】TE暖通空調(diào)系統(tǒng)高效可靠的組件解決方案
-
即日-11.13立即報名>>> 【在線會議】多物理場仿真助跑新能源汽車
-
11月28日立即報名>>> 2024工程師系列—工業(yè)電子技術(shù)在線會議
-
12月19日立即報名>> 【線下會議】OFweek 2024(第九屆)物聯(lián)網(wǎng)產(chǎn)業(yè)大會
-
即日-12.26火熱報名中>> OFweek2024中國智造CIO在線峰會
-
即日-2025.8.1立即下載>> 《2024智能制造產(chǎn)業(yè)高端化、智能化、綠色化發(fā)展藍皮書》
推薦專題
- 高級軟件工程師 廣東省/深圳市
- 自動化高級工程師 廣東省/深圳市
- 光器件研發(fā)工程師 福建省/福州市
- 銷售總監(jiān)(光器件) 北京市/海淀區(qū)
- 激光器高級銷售經(jīng)理 上海市/虹口區(qū)
- 光器件物理工程師 北京市/海淀區(qū)
- 激光研發(fā)工程師 北京市/昌平區(qū)
- 技術(shù)專家 廣東省/江門市
- 封裝工程師 北京市/海淀區(qū)
- 結(jié)構(gòu)工程師 廣東省/深圳市