使用深度學(xué)習(xí)進(jìn)行腦腫瘤檢測(cè)和定位:Part2
問(wèn)題陳述通過(guò)使用 Kaggle 的 MRI 數(shù)據(jù)集的圖像分割來(lái)預(yù)測(cè)和定位腦腫瘤。這是該系列的第二部分。如果你還沒(méi)有閱讀第一部分,我建議你訪問(wèn)使用深度學(xué)習(xí)進(jìn)行腦腫瘤檢測(cè)和定位:第1部分以更好地理解代碼,因?yàn)檫@兩個(gè)部分是相互關(guān)聯(lián)的。文章地址:https://mp.weixin.qq.com/s/vBsTsVvHjA0gtQy3X1wdmw我們?cè)?ResNet50 上訓(xùn)練了一個(gè)分類(lèi)模型,該模型使用回調(diào)對(duì)腦部 MRI 是否有腫瘤進(jìn)行分類(lèi)以提高我們的性能。在這一部分,我們將訓(xùn)練一個(gè)模型來(lái)使用圖像分割來(lái)定位腫瘤。
現(xiàn)在,讓我們開(kāi)始實(shí)施第二部分,即構(gòu)建分割模型來(lái)定位腫瘤。圖像分割的目標(biāo)是在像素級(jí)別理解圖像。它將每個(gè)像素與某個(gè)類(lèi)相關(guān)聯(lián)。圖像分割模型產(chǎn)生的輸出稱為圖像的蒙版。首先,從我們?cè)谏弦徊糠謩?chuàng)建的數(shù)據(jù)幀中選擇蒙版值為 1 的記錄,因?yàn)橹挥心[瘤存在,我們才能對(duì)其進(jìn)行定位。# Get the dataframe containing MRIs which have masks associated with them.
brain_df_mask = brain_df[brain_df['mask'] == 1]
brain_df_mask.shape
輸出:(1373, 4)將數(shù)據(jù)拆分為訓(xùn)練和測(cè)試數(shù)據(jù)集。首先,我們將整個(gè)數(shù)據(jù)拆分為訓(xùn)練和驗(yàn)證數(shù)據(jù),然后將一半的驗(yàn)證數(shù)據(jù)拆分為測(cè)試數(shù)據(jù)。from sklearn.model_selection import train_test_split
X_train, X_val = train_test_split(brain_df_mask, test_size=0.15)
X_test, X_val = train_test_split(X_val, test_size=0.5)
我們將再次使用DataGenerator 生成虛擬數(shù)據(jù),即training_generator 和validation_generator。為此,我們將首先創(chuàng)建要傳遞到生成器的圖像和蒙版路徑的列表。train_ids = list(X_train.image_path)
train_mask = list(X_train.mask_path)
val_ids = list(X_val.image_path)
val_mask= list(X_val.mask_path)
# Utilities file contains the code for custom data generator
from utilities import DataGenerator
# create image generators
training_generator = DataGenerator(train_ids,train_mask)
validation_generator = DataGenerator(val_ids,val_mask)
定義一個(gè)如下所示的方法 Resblock ,以在我們的深度學(xué)習(xí)模型中使用。模型中使用 Resblocks 以獲得更好的結(jié)果。這些塊只是一堆層。resblocks 的主要功能是在頂部學(xué)習(xí)殘差函數(shù),而信息沿底部傳遞不變。def resblock(X, f):
# make a copy of input
X_copy = X
X = Conv2D(f, kernel_size = (1,1) ,strides = (1,1),kernel_initializer ='he_normal')(X)
X = BatchNormalization()(X)
X = Activation('relu')(X)
X = Conv2D(f, kernel_size = (3,3), strides =(1,1), padding = 'same', kernel_initializer ='he_normal')(X)
X = BatchNormalization()(X)
X_copy = Conv2D(f, kernel_size = (1,1), strides =(1,1), kernel_initializer ='he_normal')(X_copy)
X_copy = BatchNormalization()(X_copy)
# Adding the output from main path and short path together
X = Add()([X,X_copy])
X = Activation('relu')(X)
return X
同樣,定義 upsample_concat 方法來(lái)放大和連接傳遞的值。Upsampling 層是一個(gè)簡(jiǎn)單的層,沒(méi)有權(quán)重,可以將輸入的維度加倍。def upsample_concat(x, skip):
x = UpSampling2D((2,2))(x)
merge = Concatenate()([x, skip])
return merge
建立一個(gè)分割模型,添加下面顯示的層,包括上面定義的 resblock 和 upsample_concat。input_shape = (256,256,3)
# Input tensor shape
X_input = Input(input_shape)
# Stage 1
conv1_in = Conv2D(16,3,activation= 'relu', padding = 'same', kernel_initializer ='he_normal')(X_input)
conv1_in = BatchNormalization()(conv1_in)
conv1_in = Conv2D(16,3,activation= 'relu', padding = 'same', kernel_initializer ='he_normal')(conv1_in)
conv1_in = BatchNormalization()(conv1_in)
pool_1 = MaxPool2D(pool_size = (2,2))(conv1_in)
# Stage 2
conv2_in = resblock(pool_1, 32)
pool_2 = MaxPool2D(pool_size = (2,2))(conv2_in)
# Stage 3
conv3_in = resblock(pool_2, 64)
pool_3 = MaxPool2D(pool_size = (2,2))(conv3_in)
# Stage 4
conv4_in = resblock(pool_3, 128)
pool_4 = MaxPool2D(pool_size = (2,2))(conv4_in)
# Stage 5 (Bottle Neck)
conv5_in = resblock(pool_4, 256)
# Upscale stage 1
up_1 = upsample_concat(conv5_in, conv4_in)
up_1 = resblock(up_1, 128)
# Upscale stage 2
up_2 = upsample_concat(up_1, conv3_in)
up_2 = resblock(up_2, 64)
# Upscale stage 3
up_3 = upsample_concat(up_2, conv2_in)
up_3 = resblock(up_3, 32)
# Upscale stage 4
up_4 = upsample_concat(up_3, conv1_in)
up_4 = resblock(up_4, 16)
# Final Output
output = Conv2D(1, (1,1), padding = "same", activation = "sigmoid")(up_4)
model_seg = Model(inputs = X_input, outputs = output )
編譯上面訓(xùn)練的模型。這次我們將自定義優(yōu)化器的參數(shù)。Focal tversky 是損失函數(shù),tversky 是度量。# Utilities file also contains the code for custom loss function
from utilities import focal_tversky, tversky
# Compile the model
adam = tf.keras.optimizers.Adam(lr = 0.05, epsilon = 0.1)
model_seg.compile(optimizer = adam, loss = focal_tversky, metrics = [tversky])
現(xiàn)在,你知道我們?cè)诜诸?lèi)器模型中使用的回調(diào)。我們將使用相同的方法來(lái)獲得更好的性能。最后,我們訓(xùn)練我們的分割模型。# use early stopping to exit training if validation loss is not decreasing even after certain epochs.
earlystopping = EarlyStopping(monitor='val_loss', mode='min', verbose=1, patience=20)
# save the best model with lower validation loss
checkpointer = ModelCheckpoint(filepath="ResUNet-weights.hdf5", verbose=1, save_best_only=True)
model_seg.fit(training_generator, epochs = 1, validation_data = validation_generator, callbacks = [checkpointer, earlystopping])
預(yù)測(cè)測(cè)試數(shù)據(jù)集的蒙版。這里,model是前面訓(xùn)練的分類(lèi)器模型,model_seg是上面訓(xùn)練的分割模型。from utilities import prediction
# making prediction
image_id, mask, has_mask = prediction(test, model, model_seg)
輸出將為我們提供圖像路徑、預(yù)測(cè)蒙版和類(lèi)標(biāo)簽。根據(jù)預(yù)測(cè)結(jié)果創(chuàng)建數(shù)據(jù)幀并與 image_path 上的測(cè)試數(shù)據(jù)幀合并。# creating a dataframe for the result
df_pred = pd.DataFrame({'image_path': image_id,'predicted_mask': mask,'has_mask': has_mask})
# Merge the dataframe containing predicted results with the original test data.
df_pred = test.merge(df_pred, on = 'image_path')
df_pred.head()
正如你在輸出中看到的那樣,我們現(xiàn)在已將最終預(yù)測(cè)的蒙版合并到我們的數(shù)據(jù)幀中。最后,將原始圖像、原始蒙版和預(yù)測(cè)蒙版一起可視化,以分析我們的分割模型的準(zhǔn)確性。count = 0
fig, axs = plt.subplots(10, 5, figsize=(30, 50))
for i in range(len(df_pred)):
if df_pred['has_mask'][i] == 1 and count < 5:
# read the images and convert them to RGB format
img = io.imread(df_pred.image_path[i])
img = cv2.cvtColor(img, cv2.COLOR_BGR2RGB)
axs[count][0].title.set_text("Brain MRI")
axs[count][0].imshow(img)
# Obtain the mask for the image
mask = io.imread(df_pred.mask_path[i])
axs[count][1].title.set_text("Original Mask")
axs[count][1].imshow(mask)
# Obtain the predicted mask for the image
predicted_mask = np.a(chǎn)sarray(df_pred.predicted_mask[i])[0].squeeze().round()
axs[count][2].title.set_text("AI Predicted Mask")
axs[count][2].imshow(predicted_mask)
# Apply the mask to the image 'mask==255'
img[mask == 255] = (255, 0, 0)
axs[count][3].title.set_text("MRI with Original Mask (Ground Truth)")
axs[count][3].imshow(img)
img_ = io.imread(df_pred.image_path[i])
img_ = cv2.cvtColor(img_, cv2.COLOR_BGR2RGB)
img_[predicted_mask == 1] = (0, 255, 0)
axs[count][4].title.set_text("MRI with AI Predicted Mask")
axs[count][4].imshow(img_)
count += 1
fig.tight_layout()
輸出顯示我們的分割模型非常好地定位了腫瘤。做得好!此外,你可以嘗試向目前訓(xùn)練的模型添加更多層并分析性能。還可以將類(lèi)似的解決方案應(yīng)用于其他問(wèn)題陳述,因?yàn)閳D像分割是當(dāng)今非常感興趣的領(lǐng)域。
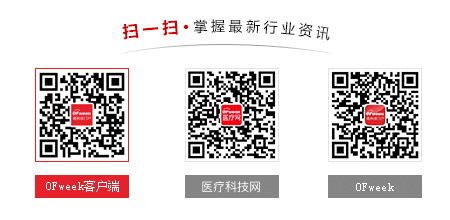
發(fā)表評(píng)論
請(qǐng)輸入評(píng)論內(nèi)容...
請(qǐng)輸入評(píng)論/評(píng)論長(zhǎng)度6~500個(gè)字
圖片新聞
-
金百澤科技亮相中國(guó)國(guó)際醫(yī)療器械博覽會(huì) | 盡顯醫(yī)療領(lǐng)域硬實(shí)力
-
進(jìn)階的新冠疫苗 又一個(gè)中國(guó)造
-
“AI醫(yī)療第一股”鷹瞳科技上市首日即破發(fā)
-
圓心科技登陸港股,“賣(mài)藥的生意”還好不好做?
-
十圖解讀2021年中國(guó)康復(fù)醫(yī)療行業(yè)現(xiàn)狀
-
醫(yī)藥流通數(shù)字化運(yùn)營(yíng)實(shí)現(xiàn)精細(xì)化飼養(yǎng)
-
科學(xué)家發(fā)現(xiàn)人體新器官:將有助于癌癥治療
-
李飛飛入選美國(guó)國(guó)家醫(yī)學(xué)院
最新活動(dòng)更多
-
11月19日立即報(bào)名>> 【線下論壇】華邦電子與恩智浦聯(lián)合技術(shù)論壇
-
11月29日立即預(yù)約>> 【上海線下】設(shè)計(jì),易如反掌—Creo 11發(fā)布巡展
-
即日-12.26火熱報(bào)名中>> OFweek2024中國(guó)智造CIO在線峰會(huì)
-
精彩回顧立即查看>> 2024(第五屆)全球數(shù)字經(jīng)濟(jì)產(chǎn)業(yè)大會(huì)暨展覽會(huì)
-
精彩回顧立即查看>> 全數(shù)會(huì)2024中國(guó)人形機(jī)器人技術(shù)創(chuàng)新發(fā)展大會(huì)
-
精彩回顧立即查看>> OFweek 2024中國(guó)激光產(chǎn)業(yè)高質(zhì)量發(fā)展峰會(huì)
-
10 BD新浪潮
- 1 南京殺出超級(jí)IPO:年入27億,華東第一
- 2 從巨額回購(gòu) 看石藥集團(tuán)的“三張”價(jià)值底牌
- 3 被華為刷屏的腦機(jī)接口芯片,有多前沿?
- 4 中國(guó)藥企出海的“PlanB”
- 5 星形膠質(zhì)細(xì)胞為阿爾茨海默病治療帶來(lái)可能
- 6 聯(lián)影醫(yī)療:貢獻(xiàn)超億元收入上演控制權(quán)迷局
- 7 慢下來(lái)的邁瑞醫(yī)療
- 8 石藥集團(tuán)的陽(yáng)謀
- 9 聯(lián)影醫(yī)療:原子公司變關(guān)聯(lián)方后或“藕斷絲連”
- 10 生物合成NMN獲突破,產(chǎn)量提升超100倍!
- 高級(jí)軟件工程師 廣東省/深圳市
- 自動(dòng)化高級(jí)工程師 廣東省/深圳市
- 光器件研發(fā)工程師 福建省/福州市
- 銷(xiāo)售總監(jiān)(光器件) 北京市/海淀區(qū)
- 激光器高級(jí)銷(xiāo)售經(jīng)理 上海市/虹口區(qū)
- 光器件物理工程師 北京市/海淀區(qū)
- 激光研發(fā)工程師 北京市/昌平區(qū)
- 技術(shù)專(zhuān)家 廣東省/江門(mén)市
- 封裝工程師 北京市/海淀區(qū)
- 結(jié)構(gòu)工程師 廣東省/深圳市