一文詳解Hive知識體系
lateral view用于和split、explode等UDTF一起使用的,能將一行數(shù)據(jù)拆分成多行數(shù)據(jù),在此基礎(chǔ)上可以對拆分的數(shù)據(jù)進(jìn)行聚合,lateral view首先為原始表的每行調(diào)用UDTF,UDTF會把一行拆分成一行或者多行,lateral view在把結(jié)果組合,產(chǎn)生一個支持別名表的虛擬表。
其中explode還可以用于將hive一列中復(fù)雜的array或者map結(jié)構(gòu)拆分成多行
需求:現(xiàn)在有數(shù)據(jù)格式如下
zhangsan child1,child2,child3,child4 k1:v1,k2:v2
lisi child5,child6,child7,child8 k3:v3,k4:v4
字段之間使用 分割,需求將所有的child進(jìn)行拆開成為一列
+----------+--+
| mychild |
+----------+--+
| child1 |
| child2 |
| child3 |
| child4 |
| child5 |
| child6 |
| child7 |
| child8 |
+----------+--+
將map的key和value也進(jìn)行拆開,成為如下結(jié)果
+-----------+-------------+--+
| mymapkey | mymapvalue |
+-----------+-------------+--+
| k1 | v1 |
| k2 | v2 |
| k3 | v3 |
| k4 | v4 |
+-----------+-------------+--+
創(chuàng)建hive數(shù)據(jù)庫創(chuàng)建hive數(shù)據(jù)庫
hive (default)> create database hive_explode;
hive (default)> use hive_explode;
創(chuàng)建hive表,然后使用explode拆分map和arrayhive (hive_explode)> create table t3(name string,children array
需求: 需求:現(xiàn)在有一些數(shù)據(jù)格式如下:
a:shandong,b:beijing,c:hebei|1,2,3,4,5,6,7,8,9|[{"source":"7fresh","monthSales":4900,"userCount":1900,"score":"9.9"},{"source":"jd","monthSales":2090,"userCount":78981,"score":"9.8"},{"source":"jdmart","monthSales":6987,"userCount":1600,"score":"9.0"}]
其中字段與字段之間的分隔符是 |
我們要解析得到所有的monthSales對應(yīng)的值為以下這一列(行轉(zhuǎn)列)
4900
2090
6987
創(chuàng)建hive表hive (hive_explode)> create table explode_lateral_view
> (`area` string,
> `goods_id` string,
> `sale_info` string)
> ROW FORMAT DELIMITED
> FIELDS TERMINATED BY '|'
> STORED AS textfile;
準(zhǔn)備數(shù)據(jù)并加載數(shù)據(jù)準(zhǔn)備數(shù)據(jù)如下
cd /export/servers/hivedatas
vim explode_json
a:shandong,b:beijing,c:hebei|1,2,3,4,5,6,7,8,9|[{"source":"7fresh","monthSales":4900,"userCount":1900,"score":"9.9"},{"source":"jd","monthSales":2090,"userCount":78981,"score":"9.8"},{"source":"jdmart","monthSales":6987,"userCount":1600,"score":"9.0"}]
加載數(shù)據(jù)到hive表當(dāng)中去
hive (hive_explode)> load data local inpath '/export/servers/hivedatas/explode_json' overwrite into table explode_lateral_view;
使用explode拆分Arrayhive (hive_explode)> select explode(split(goods_id,',')) as goods_id from explode_lateral_view;
使用explode拆解Maphive (hive_explode)> select explode(split(area,',')) as area from explode_lateral_view;
拆解json字段hive (hive_explode)> select explode(split(regexp_replace(regexp_replace(sale_info,'\[\{',''),'}]',''),'},\{')) as sale_info from explode_lateral_view;
然后我們想用get_json_object來獲取key為monthSales的數(shù)據(jù):
hive (hive_explode)> select get_json_object(explode(split(regexp_replace(regexp_replace(sale_info,'\[\{',''),'}]',''),'},\{')),'$.monthSales') as sale_info from explode_lateral_view;
然后掛了FAILED: SemanticException [Error 10081]: UDTF's are not supported outside the SELECT clause, nor nested in expressions
UDTF explode不能寫在別的函數(shù)內(nèi)
如果你這么寫,想查兩個字段,select explode(split(area,',')) as area,good_id from explode_lateral_view;
會報錯FAILED: SemanticException 1:40 Only a single expression in the SELECT clause is supported with UDTF's. Error encountered near token 'good_id'
使用UDTF的時候,只支持一個字段,這時候就需要LATERAL VIEW出場了
配合LATERAL VIEW使用
配合lateral view查詢多個字段
hive (hive_explode)> select goods_id2,sale_info from explode_lateral_view LATERAL VIEW explode(split(goods_id,','))goods as goods_id2;
其中LATERAL VIEW explode(split(goods_id,','))goods相當(dāng)于一個虛擬表,與原表explode_lateral_view笛卡爾積關(guān)聯(lián)
也可以多重使用
hive (hive_explode)> select goods_id2,sale_info,area2
from explode_lateral_view
LATERAL VIEW explode(split(goods_id,','))goods as goods_id2
LATERAL VIEW explode(split(area,','))area as area2;也是三個表笛卡爾積的結(jié)果
最終,我們可以通過下面的句子,把這個json格式的一行數(shù)據(jù),完全轉(zhuǎn)換成二維表的方式展現(xiàn)
hive (hive_explode)> select get_json_object(concat('{',sale_info_1,'}'),'$.source') as source,get_json_object(concat('{',sale_info_1,'}'),'$.monthSales') as monthSales,get_json_object(concat('{',sale_info_1,'}'),'$.userCount') as monthSales,get_json_object(concat('{',sale_info_1,'}'),'$.score') as monthSales from explode_lateral_view LATERAL VIEW explode(split(regexp_replace(regexp_replace(sale_info,'\[\{',''),'}]',''),'},\{'))sale_info as sale_info_1;
總結(jié):
Lateral View通常和UDTF一起出現(xiàn),為了解決UDTF不允許在select字段的問題。Multiple Lateral View可以實現(xiàn)類似笛卡爾乘積。Outer關(guān)鍵字可以把不輸出的UDTF的空結(jié)果,輸出成NULL,防止丟失數(shù)據(jù)。
行轉(zhuǎn)列
相關(guān)參數(shù)說明:
CONCAT(string A/col, string B/col…):返回輸入字符串連接后的結(jié)果,支持任意個輸入字符串;
CONCAT_WS(separator, str1, str2,...):它是一個特殊形式的 CONCAT()。第一個參數(shù)剩余參數(shù)間的分隔符。分隔符可以是與剩余參數(shù)一樣的字符串。如果分隔符是 NULL,返回值也將為 NULL。這個函數(shù)會跳過分隔符參數(shù)后的任何 NULL 和空字符串。分隔符將被加到被連接的字符串之間;
COLLECT_SET(col):函數(shù)只接受基本數(shù)據(jù)類型,它的主要作用是將某字段的值進(jìn)行去重匯總,產(chǎn)生array類型字段。
數(shù)據(jù)準(zhǔn)備:
nameconstellationblood_type孫悟空白羊座A老王射手座A宋宋白羊座B豬八戒白羊座A鳳姐射手座A
需求: 把星座和血型一樣的人歸類到一起。結(jié)果如下:
射手座,A 老王|鳳姐
白羊座,A 孫悟空|豬八戒
白羊座,B 宋宋
實現(xiàn)步驟:
創(chuàng)建本地constellation.txt,導(dǎo)入數(shù)據(jù)node03服務(wù)器執(zhí)行以下命令創(chuàng)建文件,注意數(shù)據(jù)使用 進(jìn)行分割
cd /export/servers/hivedatas
vim constellation.txt
數(shù)據(jù)如下:
孫悟空 白羊座 A
老王 射手座 A
宋宋 白羊座 B
豬八戒 白羊座 A
鳳姐 射手座 A
創(chuàng)建hive表并導(dǎo)入數(shù)據(jù)創(chuàng)建hive表并加載數(shù)據(jù)
hive (hive_explode)> create table person_info(
name string,
constellation string,
blood_type string)
row format delimited fields terminated by " ";
加載數(shù)據(jù)
hive (hive_explode)> load data local inpath '/export/servers/hivedatas/constellation.txt' into table person_info;
按需求查詢數(shù)據(jù)hive (hive_explode)> select
t1.base,
concat_ws('|', collect_set(t1.name)) name
from
(select
name,
concat(constellation, "," , blood_type) base
from
person_info) t1
group by
t1.base;
列轉(zhuǎn)行
所需函數(shù):
EXPLODE(col):將hive一列中復(fù)雜的array或者map結(jié)構(gòu)拆分成多行。
LATERAL VIEW
用法:LATERAL VIEW udtf(expression) tableAlias AS columnAlias
解釋:用于和split, explode等UDTF一起使用,它能夠?qū)⒁涣袛?shù)據(jù)拆成多行數(shù)據(jù),在此基礎(chǔ)上可以對拆分后的數(shù)據(jù)進(jìn)行聚合。
數(shù)據(jù)準(zhǔn)備:
cd /export/servers/hivedatas
vim movie.txt
文件內(nèi)容如下: 數(shù)據(jù)字段之間使用 進(jìn)行分割
《疑犯追蹤》 懸疑,動作,科幻,劇情
《Lie to me》 懸疑,警匪,動作,心理,劇情
《戰(zhàn)狼2》 戰(zhàn)爭,動作,災(zāi)難
需求: 將電影分類中的數(shù)組數(shù)據(jù)展開。結(jié)果如下:
《疑犯追蹤》 懸疑
《疑犯追蹤》 動作
《疑犯追蹤》 科幻
《疑犯追蹤》 劇情
《Lie to me》 懸疑
《Lie to me》 警匪
《Lie to me》 動作
《Lie to me》 心理
《Lie to me》 劇情
《戰(zhàn)狼2》 戰(zhàn)爭
《戰(zhàn)狼2》 動作
《戰(zhàn)狼2》 災(zāi)難
實現(xiàn)步驟:
創(chuàng)建hive表create table movie_info(
movie string,
category array
reflect函數(shù)可以支持在sql中調(diào)用java中的自帶函數(shù),秒殺一切udf函數(shù)。
需求1: 使用java.lang.Math當(dāng)中的Max求兩列中最大值
實現(xiàn)步驟:
創(chuàng)建hive表create table test_udf(col1 int,col2 int) row format delimited fields terminated by ',';
準(zhǔn)備數(shù)據(jù)并加載數(shù)據(jù)cd /export/servers/hivedatas
vim test_udf
文件內(nèi)容如下:
1,2
4,3
6,4
7,5
5,6
加載數(shù)據(jù)hive (hive_explode)> load data local inpath '/export/servers/hivedatas/test_udf' overwrite into table test_udf;
使用java.lang.Math當(dāng)中的Max求兩列當(dāng)中的最大值hive (hive_explode)> select reflect("java.lang.Math","max",col1,col2) from test_udf;
需求2: 文件中不同的記錄來執(zhí)行不同的java的內(nèi)置函數(shù)
實現(xiàn)步驟:
創(chuàng)建hive表hive (hive_explode)> create table test_udf2(class_name string,method_name string,col1 int , col2 int) row format delimited fields terminated by ',';
準(zhǔn)備數(shù)據(jù)cd /export/servers/hivedatas
vim test_udf2
文件內(nèi)容如下:
java.lang.Math,min,1,2
java.lang.Math,max,2,3
加載數(shù)據(jù)hive (hive_explode)> load data local inpath '/export/servers/hivedatas/test_udf2' overwrite into table test_udf2;
執(zhí)行查詢hive (hive_explode)> select reflect(class_name,method_name,col1,col2) from test_udf2;
需求3: 判斷是否為數(shù)字
實現(xiàn)方式:
使用apache commons中的函數(shù),commons下的jar已經(jīng)包含在hadoop的classpath中,所以可以直接使用。
select reflect("org.a(chǎn)pache.commons.lang.math.NumberUtils","isNumber","123")
窗口函數(shù)與分析函數(shù)
在sql中有一類函數(shù)叫做聚合函數(shù),例如sum()、avg()、max()等等,這類函數(shù)可以將多行數(shù)據(jù)按照規(guī)則聚集為一行,一般來講聚集后的行數(shù)是要少于聚集前的行數(shù)的。但是有時我們想要既顯示聚集前的數(shù)據(jù),又要顯示聚集后的數(shù)據(jù),這時我們便引入了窗口函數(shù)。窗口函數(shù)又叫OLAP函數(shù)/分析函數(shù),窗口函數(shù)兼具分組和排序功能。
窗口函數(shù)最重要的關(guān)鍵字是 partition by 和 order by。
具體語法如下:over (partition by xxx order by xxx)
sum、avg、min、max
準(zhǔn)備數(shù)據(jù)
建表語句:
create table test_t1(
cookieid string,
createtime string, --day
pv int
) row format delimited
fields terminated by ',';
加載數(shù)據(jù):
load data local inpath '/root/hivedata/test_t1.dat' into table test_t1;
cookie1,2020-04-10,1
cookie1,2020-04-11,5
cookie1,2020-04-12,7
cookie1,2020-04-13,3
cookie1,2020-04-14,2
cookie1,2020-04-15,4
cookie1,2020-04-16,4
開啟智能本地模式
SET hive.exec.mode.local.a(chǎn)uto=true;
SUM函數(shù)和窗口函數(shù)的配合使用:結(jié)果和ORDER BY相關(guān),默認(rèn)為升序。
select cookieid,createtime,pv,
sum(pv) over(partition by cookieid order by createtime) as pv1
from test_t1;
select cookieid,createtime,pv,
sum(pv) over(partition by cookieid order by createtime rows between unbounded preceding and current row) as pv2
from test_t1;
select cookieid,createtime,pv,
sum(pv) over(partition by cookieid) as pv3
from test_t1;
select cookieid,createtime,pv,
sum(pv) over(partition by cookieid order by createtime rows between 3 preceding and current row) as pv4
from test_t1;
select cookieid,createtime,pv,
sum(pv) over(partition by cookieid order by createtime rows between 3 preceding and 1 following) as pv5
from test_t1;
select cookieid,createtime,pv,
sum(pv) over(partition by cookieid order by createtime rows between current row and unbounded following) as pv6
from test_t1;
pv1: 分組內(nèi)從起點到當(dāng)前行的pv累積,如,11號的pv1=10號的pv+11號的pv, 12號=10號+11號+12號
pv2: 同pv1
pv3: 分組內(nèi)(cookie1)所有的pv累加
pv4: 分組內(nèi)當(dāng)前行+往前3行,如,11號=10號+11號, 12號=10號+11號+12號,
13號=10號+11號+12號+13號, 14號=11號+12號+13號+14號
pv5: 分組內(nèi)當(dāng)前行+往前3行+往后1行,如,14號=11號+12號+13號+14號+15號=5+7+3+2+4=21
pv6: 分組內(nèi)當(dāng)前行+往后所有行,如,13號=13號+14號+15號+16號=3+2+4+4=13,
14號=14號+15號+16號=2+4+4=10
如果不指定rows between,默認(rèn)為從起點到當(dāng)前行;
如果不指定order by,則將分組內(nèi)所有值累加;
關(guān)鍵是理解rows between含義,也叫做window子句:
preceding:往前
following:往后
current row:當(dāng)前行
unbounded:起點
unbounded preceding 表示從前面的起點
unbounded following:表示到后面的終點
AVG,MIN,MAX,和SUM用法一樣。
row_number、rank、dense_rank、ntile
準(zhǔn)備數(shù)據(jù)
cookie1,2020-04-10,1
cookie1,2020-04-11,5
cookie1,2020-04-12,7
cookie1,2020-04-13,3
cookie1,2020-04-14,2
cookie1,2020-04-15,4
cookie1,2020-04-16,4
cookie2,2020-04-10,2
cookie2,2020-04-11,3
cookie2,2020-04-12,5
cookie2,2020-04-13,6
cookie2,2020-04-14,3
cookie2,2020-04-15,9
cookie2,2020-04-16,7
CREATE TABLE test_t2 (
cookieid string,
createtime string, --day
pv INT
) ROW FORMAT DELIMITED
FIELDS TERMINATED BY ','
stored as textfile;
加載數(shù)據(jù):
load data local inpath '/root/hivedata/test_t2.dat' into table test_t2;
ROW_NUMBER()使用
ROW_NUMBER()從1開始,按照順序,生成分組內(nèi)記錄的序列。
SELECT
cookieid,
createtime,
pv,
ROW_NUMBER() OVER(PARTITION BY cookieid ORDER BY pv desc) AS rn
FROM test_t2;
RANK 和 DENSE_RANK使用
RANK() 生成數(shù)據(jù)項在分組中的排名,排名相等會在名次中留下空位 。
DENSE_RANK()生成數(shù)據(jù)項在分組中的排名,排名相等會在名次中不會留下空位。
SELECT
cookieid,
createtime,
pv,
RANK() OVER(PARTITION BY cookieid ORDER BY pv desc) AS rn1,
DENSE_RANK() OVER(PARTITION BY cookieid ORDER BY pv desc) AS rn2,
ROW_NUMBER() OVER(PARTITION BY cookieid ORDER BY pv DESC) AS rn3
FROM test_t2
WHERE cookieid = 'cookie1';
NTILE
有時會有這樣的需求:如果數(shù)據(jù)排序后分為三部分,業(yè)務(wù)人員只關(guān)心其中的一部分,如何將這中間的三分之一數(shù)據(jù)拿出來呢?NTILE函數(shù)即可以滿足。
ntile可以看成是:把有序的數(shù)據(jù)集合平均分配到指定的數(shù)量(num)個桶中, 將桶號分配給每一行。如果不能平均分配,則優(yōu)先分配較小編號的桶,并且各個桶中能放的行數(shù)最多相差1。
然后可以根據(jù)桶號,選取前或后 n分之幾的數(shù)據(jù)。數(shù)據(jù)會完整展示出來,只是給相應(yīng)的數(shù)據(jù)打標(biāo)簽;具體要取幾分之幾的數(shù)據(jù),需要再嵌套一層根據(jù)標(biāo)簽取出。
SELECT
cookieid,
createtime,
pv,
NTILE(2) OVER(PARTITION BY cookieid ORDER BY createtime) AS rn1,
NTILE(3) OVER(PARTITION BY cookieid ORDER BY createtime) AS rn2,
NTILE(4) OVER(ORDER BY createtime) AS rn3
FROM test_t2
ORDER BY cookieid,createtime;
其他一些窗口函數(shù)lag,lead,first_value,last_valueLAG
LAG(col,n,DEFAULT) 用于統(tǒng)計窗口內(nèi)往上第n行值第一個參數(shù)為列名,第二個參數(shù)為往上第n行(可選,默認(rèn)為1),第三個參數(shù)為默認(rèn)值(當(dāng)往上第n行為NULL時候,取默認(rèn)值,如不指定,則為NULL)SELECT cookieid,
createtime,
url,
ROW_NUMBER() OVER(PARTITION BY cookieid ORDER BY createtime) AS rn,
LAG(createtime,1,'1970-01-01 00:00:00') OVER(PARTITION BY cookieid ORDER BY createtime) AS last_1_time,
LAG(createtime,2) OVER(PARTITION BY cookieid ORDER BY createtime) AS last_2_time
FROM test_t4;
last_1_time: 指定了往上第1行的值,default為'1970-01-01 00:00:00'
cookie1第一行,往上1行為NULL,因此取默認(rèn)值 1970-01-01 00:00:00
cookie1第三行,往上1行值為第二行值,2015-04-10 10:00:02
cookie1第六行,往上1行值為第五行值,2015-04-10 10:50:01
last_2_time: 指定了往上第2行的值,為指定默認(rèn)值
cookie1第一行,往上2行為NULL
cookie1第二行,往上2行為NULL
cookie1第四行,往上2行為第二行值,2015-04-10 10:00:02
cookie1第七行,往上2行為第五行值,2015-04-10 10:50:01
LEAD
與LAG相反LEAD(col,n,DEFAULT) 用于統(tǒng)計窗口內(nèi)往下第n行值第一個參數(shù)為列名,第二個參數(shù)為往下第n行(可選,默認(rèn)為1),第三個參數(shù)為默認(rèn)值(當(dāng)往下第n行為NULL時候,取默認(rèn)值,如不指定,則為NULL)
SELECT cookieid,
createtime,
url,
ROW_NUMBER() OVER(PARTITION BY cookieid ORDER BY createtime) AS rn,
LEAD(createtime,1,'1970-01-01 00:00:00') OVER(PARTITION BY cookieid ORDER BY createtime) AS next_1_time,
LEAD(createtime,2) OVER(PARTITION BY cookieid ORDER BY createtime) AS next_2_time
FROM test_t4;
FIRST_VALUE
取分組內(nèi)排序后,截止到當(dāng)前行,第一個值
SELECT cookieid,
createtime,
url,
ROW_NUMBER() OVER(PARTITION BY cookieid ORDER BY createtime) AS rn,
FIRST_VALUE(url) OVER(PARTITION BY cookieid ORDER BY createtime) AS first1
FROM test_t4;
LAST_VALUE
取分組內(nèi)排序后,截止到當(dāng)前行,最后一個值
SELECT cookieid,
createtime,
url,
ROW_NUMBER() OVER(PARTITION BY cookieid ORDER BY createtime) AS rn,
LAST_VALUE(url) OVER(PARTITION BY cookieid ORDER BY createtime) AS last1
FROM test_t4;
如果想要取分組內(nèi)排序后最后一個值,則需要變通一下:
SELECT cookieid,
createtime,
url,
ROW_NUMBER() OVER(PARTITION BY cookieid ORDER BY createtime) AS rn,
LAST_VALUE(url) OVER(PARTITION BY cookieid ORDER BY createtime) AS last1,
FIRST_VALUE(url) OVER(PARTITION BY cookieid ORDER BY createtime DESC) AS last2
FROM test_t4
ORDER BY cookieid,createtime;
特別注意order by
如果不指定ORDER BY,則進(jìn)行排序混亂,會出現(xiàn)錯誤的結(jié)果
SELECT cookieid,
createtime,
url,
FIRST_VALUE(url) OVER(PARTITION BY cookieid) AS first2
FROM test_t4;
cume_dist,percent_rank
這兩個序列分析函數(shù)不是很常用,注意:序列函數(shù)不支持WINDOW子句
數(shù)據(jù)準(zhǔn)備d1,user1,1000
d1,user2,2000
d1,user3,3000
d2,user4,4000
d2,user5,5000
CREATE EXTERNAL TABLE test_t3 (
dept STRING,
userid string,
sal INT
) ROW FORMAT DELIMITED
FIELDS TERMINATED BY ','
stored as textfile;
加載數(shù)據(jù):
load data local inpath '/root/hivedata/test_t3.dat' into table test_t3;
CUME_DIST 和order byd的排序順序有關(guān)系
CUME_DIST 小于等于當(dāng)前值的行數(shù)/分組內(nèi)總行數(shù) order 默認(rèn)順序 正序 升序比如,統(tǒng)計小于等于當(dāng)前薪水的人數(shù),所占總?cè)藬?shù)的比例
SELECT
dept,
userid,
sal,
CUME_DIST() OVER(ORDER BY sal) AS rn1,
CUME_DIST() OVER(PARTITION BY dept ORDER BY sal) AS rn2
FROM test_t3;
rn1: 沒有partition,所有數(shù)據(jù)均為1組,總行數(shù)為5,
第一行:小于等于1000的行數(shù)為1,因此,1/5=0.2
第三行:小于等于3000的行數(shù)為3,因此,3/5=0.6
rn2: 按照部門分組,dpet=d1的行數(shù)為3,
第二行:小于等于2000的行數(shù)為2,因此,2/3=0.6666666666666666
PERCENT_RANK
PERCENT_RANK 分組內(nèi)當(dāng)前行的RANK值-1/分組內(nèi)總行數(shù)-1
經(jīng)調(diào)研 該函數(shù)顯示現(xiàn)實意義不明朗 有待于繼續(xù)考證
SELECT
dept,
userid,
sal,
PERCENT_RANK() OVER(ORDER BY sal) AS rn1, --分組內(nèi)
RANK() OVER(ORDER BY sal) AS rn11, --分組內(nèi)RANK值
SUM(1) OVER(PARTITION BY NULL) AS rn12, --分組內(nèi)總行數(shù)
PERCENT_RANK() OVER(PARTITION BY dept ORDER BY sal) AS rn2
FROM test_t3;
rn1: rn1 = (rn11-1) / (rn12-1)
第一行,(1-1)/(5-1)=0/4=0
第二行,(2-1)/(5-1)=1/4=0.25
第四行,(4-1)/(5-1)=3/4=0.75
rn2: 按照dept分組,
dept=d1的總行數(shù)為3
第一行,(1-1)/(3-1)=0
第三行,(3-1)/(3-1)=1
grouping sets,grouping__id,cube,rollup
這幾個分析函數(shù)通常用于OLAP中,不能累加,而且需要根據(jù)不同維度上鉆和下鉆的指標(biāo)統(tǒng)計,比如,分小時、天、月的UV數(shù)。
數(shù)據(jù)準(zhǔn)備2020-03,2020-03-10,cookie1
2020-03,2020-03-10,cookie5
2020-03,2020-03-12,cookie7
2020-04,2020-04-12,cookie3
2020-04,2020-04-13,cookie2
2020-04,2020-04-13,cookie4
2020-04,2020-04-16,cookie4
2020-03,2020-03-10,cookie2
2020-03,2020-03-10,cookie3
2020-04,2020-04-12,cookie5
2020-04,2020-04-13,cookie6
2020-04,2020-04-15,cookie3
2020-04,2020-04-15,cookie2
2020-04,2020-04-16,cookie1
CREATE TABLE test_t5 (
month STRING,
day STRING,
cookieid STRING
) ROW FORMAT DELIMITED
FIELDS TERMINATED BY ','
stored as textfile;
加載數(shù)據(jù):
load data local inpath '/root/hivedata/test_t5.dat' into table test_t5;
GROUPING SETS
grouping sets是一種將多個group by 邏輯寫在一個sql語句中的便利寫法。
等價于將不同維度的GROUP BY結(jié)果集進(jìn)行UNION ALL。
GROUPING__ID,表示結(jié)果屬于哪一個分組集合。
SELECT
month,
day,
COUNT(DISTINCT cookieid) AS uv,
GROUPING__ID
FROM test_t5
GROUP BY month,day
GROUPING SETS (month,day)
ORDER BY GROUPING__ID;
grouping_id表示這一組結(jié)果屬于哪個分組集合,
根據(jù)grouping sets中的分組條件month,day,1是代表month,2是代表day
等價于
SELECT month,NULL,COUNT(DISTINCT cookieid) AS uv,1 AS GROUPING__ID FROM test_t5 GROUP BY month UNION ALL
SELECT NULL as month,day,COUNT(DISTINCT cookieid) AS uv,2 AS GROUPING__ID FROM test_t5 GROUP BY day;
再如:
SELECT
month,
day,
COUNT(DISTINCT cookieid) AS uv,
GROUPING__ID
FROM test_t5
GROUP BY month,day
GROUPING SETS (month,day,(month,day))
ORDER BY GROUPING__ID;
等價于
SELECT month,NULL,COUNT(DISTINCT cookieid) AS uv,1 AS GROUPING__ID FROM test_t5 GROUP BY month
UNION ALL
SELECT NULL,day,COUNT(DISTINCT cookieid) AS uv,2 AS GROUPING__ID FROM test_t5 GROUP BY day
UNION ALL
SELECT month,day,COUNT(DISTINCT cookieid) AS uv,3 AS GROUPING__ID FROM test_t5 GROUP BY month,day;
CUBE
根據(jù)GROUP BY的維度的所有組合進(jìn)行聚合。
SELECT
month,
day,
COUNT(DISTINCT cookieid) AS uv,
GROUPING__ID
FROM test_t5
GROUP BY month,day
WITH CUBE
ORDER BY GROUPING__ID;
等價于
SELECT NULL,NULL,COUNT(DISTINCT cookieid) AS uv,0 AS GROUPING__ID FROM test_t5
UNION ALL
SELECT month,NULL,COUNT(DISTINCT cookieid) AS uv,1 AS GROUPING__ID FROM test_t5 GROUP BY month
UNION ALL
SELECT NULL,day,COUNT(DISTINCT cookieid) AS uv,2 AS GROUPING__ID FROM test_t5 GROUP BY day
UNION ALL
SELECT month,day,COUNT(DISTINCT cookieid) AS uv,3 AS GROUPING__ID FROM test_t5 GROUP BY month,day;
ROLLUP
是CUBE的子集,以最左側(cè)的維度為主,從該維度進(jìn)行層級聚合。
比如,以month維度進(jìn)行層級聚合:
SELECT
month,
day,
COUNT(DISTINCT cookieid) AS uv,
GROUPING__ID
FROM test_t5
GROUP BY month,day
WITH ROLLUP
ORDER BY GROUPING__ID;
--把month和day調(diào)換順序,則以day維度進(jìn)行層級聚合:
SELECT
day,
month,
COUNT(DISTINCT cookieid) AS uv,
GROUPING__ID
FROM test_t5
GROUP BY day,month
WITH ROLLUP
ORDER BY GROUPING__ID;
(這里,根據(jù)天和月進(jìn)行聚合,和根據(jù)天聚合結(jié)果一樣,因為有父子關(guān)系,如果是其他維度組合的話,就會不一樣)
七、Hive執(zhí)行計劃
Hive SQL的執(zhí)行計劃描述SQL實際執(zhí)行的整體輪廓,通過執(zhí)行計劃能了解SQL程序在轉(zhuǎn)換成相應(yīng)計算引擎的執(zhí)行邏輯,掌握了執(zhí)行邏輯也就能更好地把握程序出現(xiàn)的瓶頸點,從而能夠?qū)崿F(xiàn)更有針對性的優(yōu)化。此外還能幫助開發(fā)者識別看似等價的SQL其實是不等價的,看似不等價的SQL其實是等價的SQL。可以說執(zhí)行計劃是打開SQL優(yōu)化大門的一把鑰匙。
要想學(xué)SQL執(zhí)行計劃,就需要學(xué)習(xí)查看執(zhí)行計劃的命令:explain,在查詢語句的SQL前面加上關(guān)鍵字explain是查看執(zhí)行計劃的基本方法。
學(xué)會explain,能夠給我們工作中使用hive帶來極大的便利!
查看SQL的執(zhí)行計劃
Hive提供的執(zhí)行計劃目前可以查看的信息有以下幾種:
explain:查看執(zhí)行計劃的基本信息;
explain dependency:dependency在explain語句中使用會產(chǎn)生有關(guān)計劃中輸入的額外信息。它顯示了輸入的各種屬性;
explain authorization:查看SQL操作相關(guān)權(quán)限的信息;
explain vectorization:查看SQL的向量化描述信息,顯示為什么未對Map和Reduce進(jìn)行矢量化。從 Hive 2.3.0 開始支持;
explain analyze:用實際的行數(shù)注釋計劃。從 Hive 2.2.0 開始支持;
explain cbo:輸出由Calcite優(yōu)化器生成的計劃。CBO 從 Hive 4.0.0 版本開始支持;
explain locks:這對于了解系統(tǒng)將獲得哪些鎖以運(yùn)行指定的查詢很有用。LOCKS 從 Hive 3.2.0 開始支持;
explain ast:輸出查詢的抽象語法樹。AST 在 Hive 2.1.0 版本刪除了,存在bug,轉(zhuǎn)儲AST可能會導(dǎo)致OOM錯誤,將在4.0.0版本修復(fù);
explain extended:加上 extended 可以輸出有關(guān)計劃的額外信息。這通常是物理信息,例如文件名,這些額外信息對我們用處不大;
1. explain 的用法
Hive提供了explain命令來展示一個查詢的執(zhí)行計劃,這個執(zhí)行計劃對于我們了解底層原理,Hive 調(diào)優(yōu),排查數(shù)據(jù)傾斜等很有幫助。
使用語法如下:
explain query;
在 hive cli 中輸入以下命令(hive 2.3.7):
explain select sum(id) from test1;
得到結(jié)果:
STAGE DEPENDENCIES:
Stage-1 is a root stage
Stage-0 depends on stages: Stage-1
STAGE PLANS:
Stage: Stage-1
Map Reduce
Map Operator Tree:
TableScan
alias: test1
Statistics: Num rows: 6 Data size: 75 Basic stats: COMPLETE Column stats: NONE
Select Operator
expressions: id (type: int)
outputColumnNames: id
Statistics: Num rows: 6 Data size: 75 Basic stats: COMPLETE Column stats: NONE
Group By Operator
aggregations: sum(id)
mode: hash
outputColumnNames: _col0
Statistics: Num rows: 1 Data size: 8 Basic stats: COMPLETE Column stats: NONE
Reduce Output Operator
sort order:
Statistics: Num rows: 1 Data size: 8 Basic stats: COMPLETE Column stats: NONE
value expressions: _col0 (type: bigint)
Reduce Operator Tree:
Group By Operator
aggregations: sum(VALUE._col0)
mode: mergepartial
outputColumnNames: _col0
Statistics: Num rows: 1 Data size: 8 Basic stats: COMPLETE Column stats: NONE
File Output Operator
compressed: false
Statistics: Num rows: 1 Data size: 8 Basic stats: COMPLETE Column stats: NONE
table:
input format: org.a(chǎn)pache.hadoop.mapred.SequenceFileInputFormat
output format: org.a(chǎn)pache.hadoop.hive.ql.io.HiveSequenceFileOutputFormat
serde: org.a(chǎn)pache.hadoop.hive.serde2.lazy.LazySimpleSerDe
Stage: Stage-0
Fetch Operator
limit: -1
Processor Tree:
ListSink
看完以上內(nèi)容有什么感受,是不是感覺都看不懂,不要著急,下面將會詳細(xì)講解每個參數(shù),相信你學(xué)完下面的內(nèi)容之后再看 explain 的查詢結(jié)果將游刃有余。
一個HIVE查詢被轉(zhuǎn)換為一個由一個或多個stage組成的序列(有向無環(huán)圖DAG)。這些stage可以是MapReduce stage,也可以是負(fù)責(zé)元數(shù)據(jù)存儲的stage,也可以是負(fù)責(zé)文件系統(tǒng)的操作(比如移動和重命名)的stage。
我們將上述結(jié)果拆分看,先從最外層開始,包含兩個大的部分:
stage dependencies:各個stage之間的依賴性stage plan:各個stage的執(zhí)行計劃
先看第一部分 stage dependencies ,包含兩個 stage,Stage-1 是根stage,說明這是開始的stage,Stage-0 依賴 Stage-1,Stage-1執(zhí)行完成后執(zhí)行Stage-0。
再看第二部分 stage plan,里面有一個 Map Reduce,一個MR的執(zhí)行計劃分為兩個部分:
Map Operator Tree:MAP端的執(zhí)行計劃樹Reduce Operator Tree:Reduce端的執(zhí)行計劃樹
這兩個執(zhí)行計劃樹里面包含這條sql語句的 operator:
TableScan:表掃描操作,map端第一個操作肯定是加載表,所以就是表掃描操作,常見的屬性:alias:表名稱Statistics:表統(tǒng)計信息,包含表中數(shù)據(jù)條數(shù),數(shù)據(jù)大小等Select Operator:選取操作,常見的屬性 :expressions:需要的字段名稱及字段類型outputColumnNames:輸出的列名稱Statistics:表統(tǒng)計信息,包含表中數(shù)據(jù)條數(shù),數(shù)據(jù)大小等Group By Operator:分組聚合操作,常見的屬性:aggregations:顯示聚合函數(shù)信息mode:聚合模式,值有 hash:隨機(jī)聚合,就是hash partition;partial:局部聚合;final:最終聚合keys:分組的字段,如果沒有分組,則沒有此字段outputColumnNames:聚合之后輸出列名Statistics:表統(tǒng)計信息,包含分組聚合之后的數(shù)據(jù)條數(shù),數(shù)據(jù)大小等Reduce Output Operator:輸出到reduce操作,常見屬性:sort order:值為空 不排序;值為 + 正序排序,值為 - 倒序排序;值為 +- 排序的列為兩列,第一列為正序,第二列為倒序Filter Operator:過濾操作,常見的屬性:predicate:過濾條件,如sql語句中的where id>=1,則此處顯示(id >= 1)Map Join Operator:join 操作,常見的屬性:condition map:join方式 ,如Inner Join 0 to 1 Left Outer Join0 to 2keys: join 的條件字段outputColumnNames:join 完成之后輸出的字段Statistics:join 完成之后生成的數(shù)據(jù)條數(shù),大小等File Output Operator:文件輸出操作,常見的屬性compressed:是否壓縮table:表的信息,包含輸入輸出文件格式化方式,序列化方式等Fetch Operator 客戶端獲取數(shù)據(jù)操作,常見的屬性:limit,值為 -1 表示不限制條數(shù),其他值為限制的條數(shù)2. explain 的使用場景
本節(jié)介紹 explain 能夠為我們在生產(chǎn)實踐中帶來哪些便利及解決我們哪些迷惑
案例一:join 語句會過濾 null 的值嗎?
現(xiàn)在,我們在hive cli 輸入以下查詢計劃語句
select a.id,b.user_name from test1 a join test2 b on a.id=b.id;
問:上面這條 join 語句會過濾 id 為 null 的值嗎
執(zhí)行下面語句:
explain select a.id,b.user_name from test1 a join test2 b on a.id=b.id;
我們來看結(jié)果 (為了適應(yīng)頁面展示,僅截取了部分輸出信息):
TableScan
alias: a
Statistics: Num rows: 6 Data size: 75 Basic stats: COMPLETE Column stats: NONE
Filter Operator
predicate: id is not null (type: boolean)
Statistics: Num rows: 6 Data size: 75 Basic stats: COMPLETE Column stats: NONE
Select Operator
expressions: id (type: int)
outputColumnNames: _col0
Statistics: Num rows: 6 Data size: 75 Basic stats: COMPLETE Column stats: NONE
HashTable Sink Operator
keys:
0 _col0 (type: int)
1 _col0 (type: int)
...
從上述結(jié)果可以看到 predicate: id is not null 這樣一行,說明 join 時會自動過濾掉關(guān)聯(lián)字段為 null值的情況,但 left join 或 full join 是不會自動過濾null值的,大家可以自行嘗試下。
案例二:group by 分組語句會進(jìn)行排序嗎?
看下面這條sql
select id,max(user_name) from test1 group by id;
問:group by 分組語句會進(jìn)行排序嗎
直接來看 explain 之后結(jié)果 (為了適應(yīng)頁面展示,僅截取了部分輸出信息)
TableScan
alias: test1
Statistics: Num rows: 9 Data size: 108 Basic stats: COMPLETE Column stats: NONE
Select Operator
expressions: id (type: int), user_name (type: string)
outputColumnNames: id, user_name
Statistics: Num rows: 9 Data size: 108 Basic stats: COMPLETE Column stats: NONE
Group By Operator
aggregations: max(user_name)
keys: id (type: int)
mode: hash
outputColumnNames: _col0, _col1
Statistics: Num rows: 9 Data size: 108 Basic stats: COMPLETE Column stats: NONE
Reduce Output Operator
key expressions: _col0 (type: int)
sort order: +
Map-reduce partition columns: _col0 (type: int)
Statistics: Num rows: 9 Data size: 108 Basic stats: COMPLETE Column stats: NONE
value expressions: _col1 (type: string)
...
我們看 Group By Operator,里面有 keys: id (type: int) 說明按照 id 進(jìn)行分組的,再往下看還有 sort order: + ,說明是按照 id 字段進(jìn)行正序排序的。
案例三:哪條sql執(zhí)行效率高呢?
觀察兩條sql語句
SELECT
a.id,
b.user_name
FROM
test1 a
JOIN test2 b ON a.id = b.id
WHERE
a.id > 2;
SELECT
a.id,
b.user_name
FROM
(SELECT * FROM test1 WHERE id > 2) a
JOIN test2 b ON a.id = b.id;
這兩條sql語句輸出的結(jié)果是一樣的,但是哪條sql執(zhí)行效率高呢?
有人說第一條sql執(zhí)行效率高,因為第二條sql有子查詢,子查詢會影響性能;
有人說第二條sql執(zhí)行效率高,因為先過濾之后,在進(jìn)行join時的條數(shù)減少了,所以執(zhí)行效率就高了。
到底哪條sql效率高呢,我們直接在sql語句前面加上 explain,看下執(zhí)行計劃不就知道了嘛!
在第一條sql語句前加上 explain,得到如下結(jié)果
hive (default)> explain select a.id,b.user_name from test1 a join test2 b on a.id=b.id where a.id >2;
OK
Explain
STAGE DEPENDENCIES:
Stage-4 is a root stage
Stage-3 depends on stages: Stage-4
Stage-0 depends on stages: Stage-3
STAGE PLANS:
Stage: Stage-4
Map Reduce Local Work
Alias -> Map Local Tables:
$hdt$_0:a
Fetch Operator
limit: -1
Alias -> Map Local Operator Tree:
$hdt$_0:a
TableScan
alias: a
Statistics: Num rows: 6 Data size: 75 Basic stats: COMPLETE Column stats: NONE
Filter Operator
predicate: (id > 2) (type: boolean)
Statistics: Num rows: 2 Data size: 25 Basic stats: COMPLETE Column stats: NONE
Select Operator
expressions: id (type: int)
outputColumnNames: _col0
Statistics: Num rows: 2 Data size: 25 Basic stats: COMPLETE Column stats: NONE
HashTable Sink Operator
keys:
0 _col0 (type: int)
1 _col0 (type: int)
Stage: Stage-3
Map Reduce
Map Operator Tree:
TableScan
alias: b
Statistics: Num rows: 6 Data size: 75 Basic stats: COMPLETE Column stats: NONE
Filter Operator
predicate: (id > 2) (type: boolean)
Statistics: Num rows: 2 Data size: 25 Basic stats: COMPLETE Column stats: NONE
Select Operator
expressions: id (type: int), user_name (type: string)
outputColumnNames: _col0, _col1
Statistics: Num rows: 2 Data size: 25 Basic stats: COMPLETE Column stats: NONE
Map Join Operator
condition map:
Inner Join 0 to 1
keys:
0 _col0 (type: int)
1 _col0 (type: int)
outputColumnNames: _col0, _col2
Statistics: Num rows: 2 Data size: 27 Basic stats: COMPLETE Column stats: NONE
Select Operator
expressions: _col0 (type: int), _col2 (type: string)
outputColumnNames: _col0, _col1
Statistics: Num rows: 2 Data size: 27 Basic stats: COMPLETE Column stats: NONE
File Output Operator
compressed: false
Statistics: Num rows: 2 Data size: 27 Basic stats: COMPLETE Column stats: NONE
table:
input format: org.a(chǎn)pache.hadoop.mapred.SequenceFileInputFormat
output format: org.a(chǎn)pache.hadoop.hive.ql.io.HiveSequenceFileOutputFormat
serde: org.a(chǎn)pache.hadoop.hive.serde2.lazy.LazySimpleSerDe
Local Work:
Map Reduce Local Work
Stage: Stage-0
Fetch Operator
limit: -1
Processor Tree:
ListSink
在第二條sql語句前加上 explain,得到如下結(jié)果
hive (default)> explain select a.id,b.user_name from(select * from test1 where id>2 ) a join test2 b on a.id=b.id;
OK
Explain
STAGE DEPENDENCIES:
Stage-4 is a root stage
Stage-3 depends on stages: Stage-4
Stage-0 depends on stages: Stage-3
STAGE PLANS:
Stage: Stage-4
Map Reduce Local Work
Alias -> Map Local Tables:
$hdt$_0:test1
Fetch Operator
limit: -1
Alias -> Map Local Operator Tree:
$hdt$_0:test1
TableScan
alias: test1
Statistics: Num rows: 6 Data size: 75 Basic stats: COMPLETE Column stats: NONE
Filter Operator
predicate: (id > 2) (type: boolean)
Statistics: Num rows: 2 Data size: 25 Basic stats: COMPLETE Column stats: NONE
Select Operator
expressions: id (type: int)
outputColumnNames: _col0
Statistics: Num rows: 2 Data size: 25 Basic stats: COMPLETE Column stats: NONE
HashTable Sink Operator
keys:
0 _col0 (type: int)
1 _col0 (type: int)
Stage: Stage-3
Map Reduce
Map Operator Tree:
TableScan
alias: b
Statistics: Num rows: 6 Data size: 75 Basic stats: COMPLETE Column stats: NONE
Filter Operator
predicate: (id > 2) (type: boolean)
Statistics: Num rows: 2 Data size: 25 Basic stats: COMPLETE Column stats: NONE
Select Operator
expressions: id (type: int), user_name (type: string)
outputColumnNames: _col0, _col1
Statistics: Num rows: 2 Data size: 25 Basic stats: COMPLETE Column stats: NONE
Map Join Operator
condition map:
Inner Join 0 to 1
keys:
0 _col0 (type: int)
1 _col0 (type: int)
outputColumnNames: _col0, _col2
Statistics: Num rows: 2 Data size: 27 Basic stats: COMPLETE Column stats: NONE
Select Operator
expressions: _col0 (type: int), _col2 (type: string)
outputColumnNames: _col0, _col1
Statistics: Num rows: 2 Data size: 27 Basic stats: COMPLETE Column stats: NONE
File Output Operator
compressed: false
Statistics: Num rows: 2 Data size: 27 Basic stats: COMPLETE Column stats: NONE
table:
input format: org.a(chǎn)pache.hadoop.mapred.SequenceFileInputFormat
output format: org.a(chǎn)pache.hadoop.hive.ql.io.HiveSequenceFileOutputFormat
serde: org.a(chǎn)pache.hadoop.hive.serde2.lazy.LazySimpleSerDe
Local Work:
Map Reduce Local Work
Stage: Stage-0
Fetch Operator
limit: -1
Processor Tree:
ListSink
大家有什么發(fā)現(xiàn),除了表別名不一樣,其他的執(zhí)行計劃完全一樣,都是先進(jìn)行 where 條件過濾,在進(jìn)行 join 條件關(guān)聯(lián)。說明 hive 底層會自動幫我們進(jìn)行優(yōu)化,所以這兩條sql語句執(zhí)行效率是一樣的。
以上僅列舉了3個我們生產(chǎn)中既熟悉又有點迷糊的例子,explain 還有很多其他的用途,如查看stage的依賴情況、排查數(shù)據(jù)傾斜、hive 調(diào)優(yōu)等,小伙伴們可以自行嘗試。
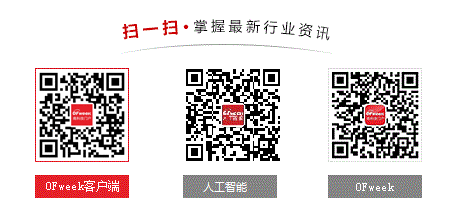
請輸入評論內(nèi)容...
請輸入評論/評論長度6~500個字
最新活動更多
-
即日-11.13立即報名>>> 【在線會議】多物理場仿真助跑新能源汽車
-
11月28日立即報名>>> 2024工程師系列—工業(yè)電子技術(shù)在線會議
-
12月19日立即報名>> 【線下會議】OFweek 2024(第九屆)物聯(lián)網(wǎng)產(chǎn)業(yè)大會
-
即日-12.26火熱報名中>> OFweek2024中國智造CIO在線峰會
-
即日-2025.8.1立即下載>> 《2024智能制造產(chǎn)業(yè)高端化、智能化、綠色化發(fā)展藍(lán)皮書》
-
精彩回顧立即查看>> 【限時免費(fèi)下載】TE暖通空調(diào)系統(tǒng)高效可靠的組件解決方案
推薦專題
- 高級軟件工程師 廣東省/深圳市
- 自動化高級工程師 廣東省/深圳市
- 光器件研發(fā)工程師 福建省/福州市
- 銷售總監(jiān)(光器件) 北京市/海淀區(qū)
- 激光器高級銷售經(jīng)理 上海市/虹口區(qū)
- 光器件物理工程師 北京市/海淀區(qū)
- 激光研發(fā)工程師 北京市/昌平區(qū)
- 技術(shù)專家 廣東省/江門市
- 封裝工程師 北京市/海淀區(qū)
- 結(jié)構(gòu)工程師 廣東省/深圳市